Well, we’re between coup attempts and attempts to throw out a seditionist president, so I’m going to take the opportunity to describe this research that was recently accepted in @SERestoration for the grassland ecology SF.
New #RestorationEcology article! https://t.co/lEKdclptYc
— SERestoration (@SERestoration) December 23, 2020
"This study provides a path toward a new level of ease and precision in monitoring community dynamics of restored grasslands." \U0001f33f pic.twitter.com/kM7L9y2jrR
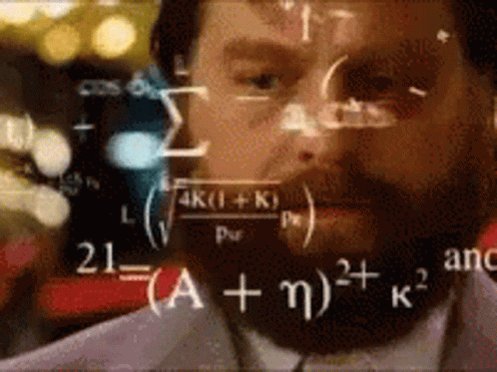
Parrot provided this drone to us via a climate change grant (thanks, Parrot!).
Here is how takeoffs are supposed to go, btw.
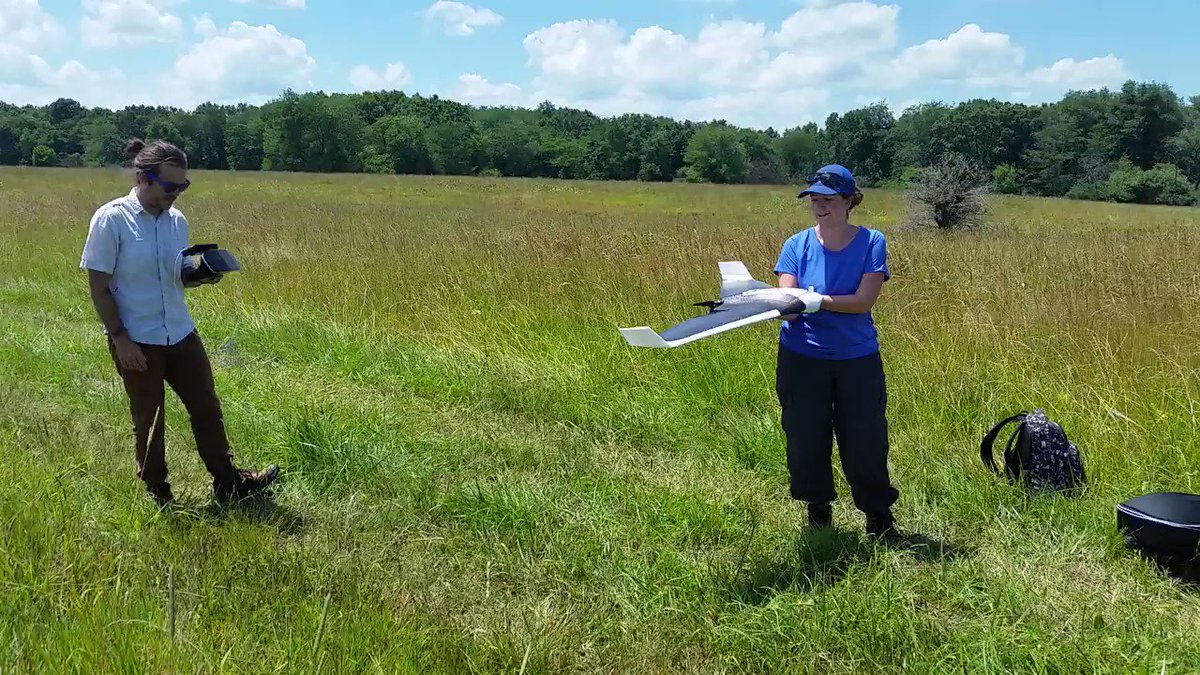
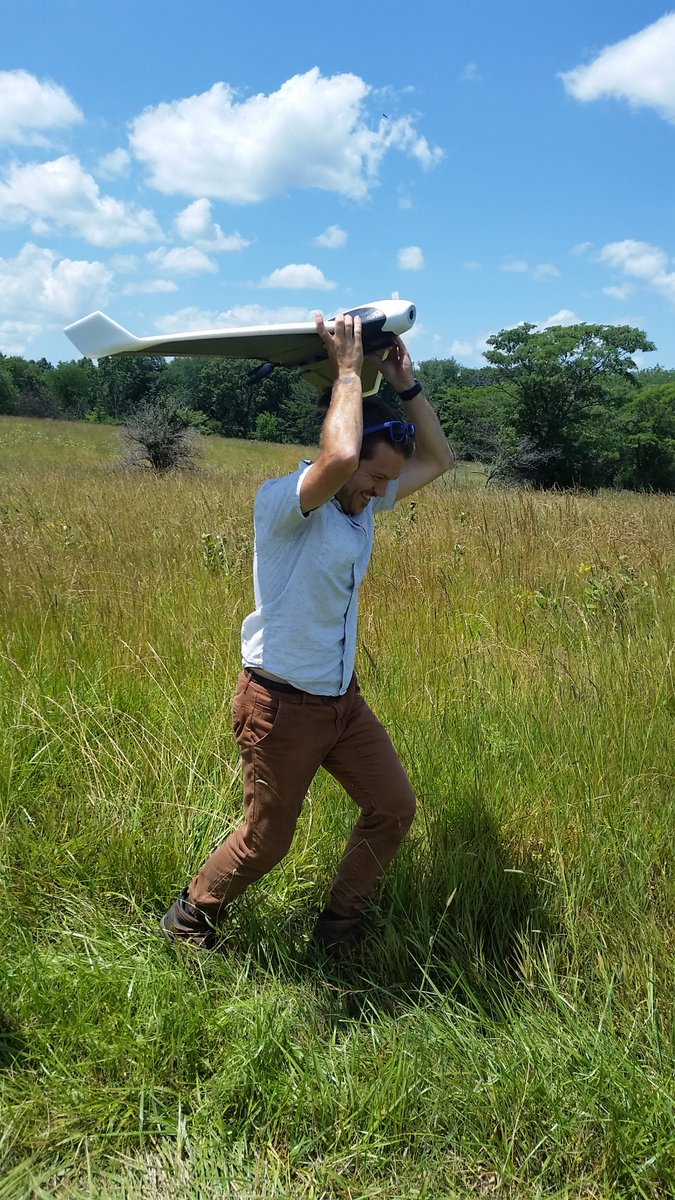
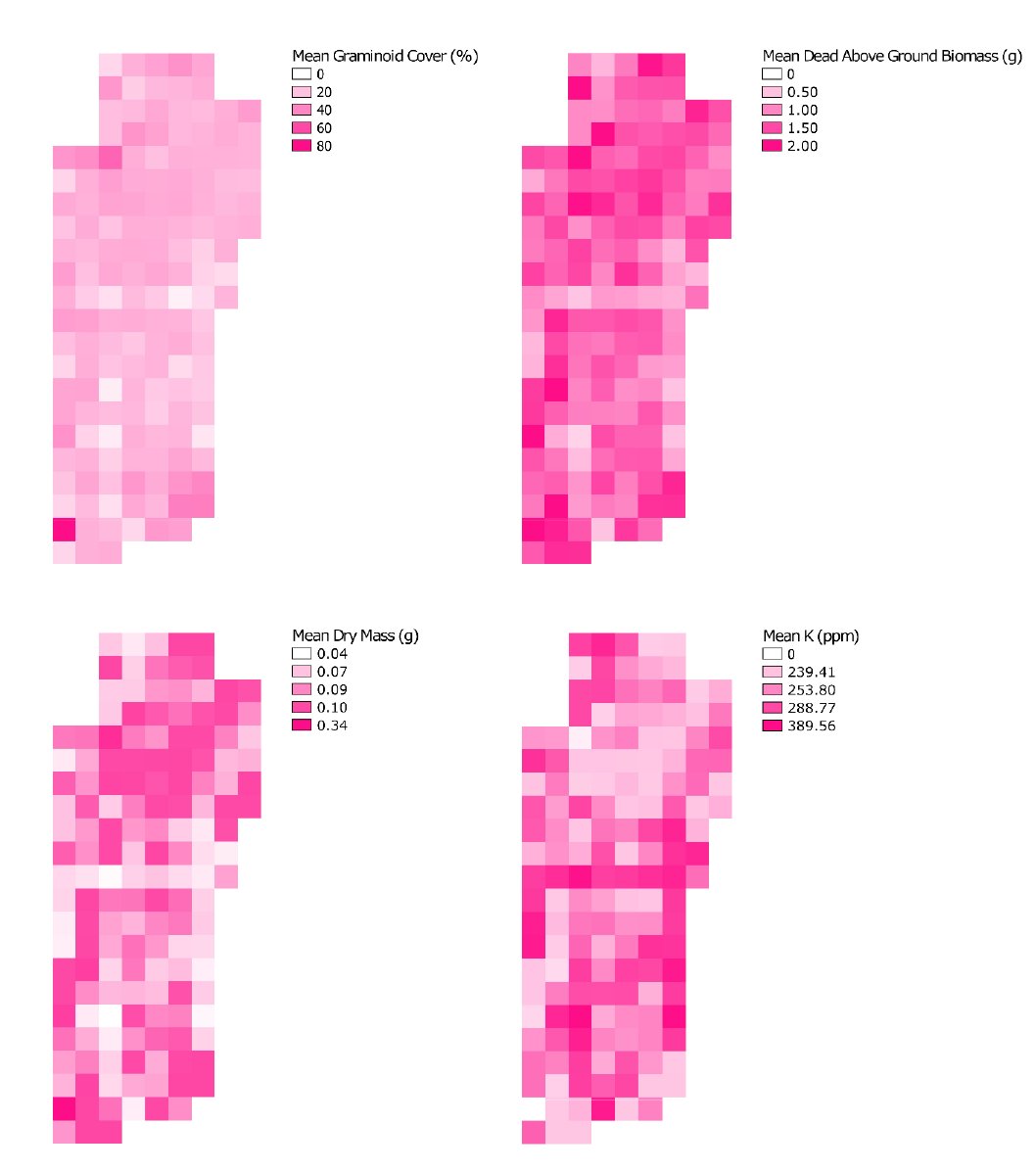
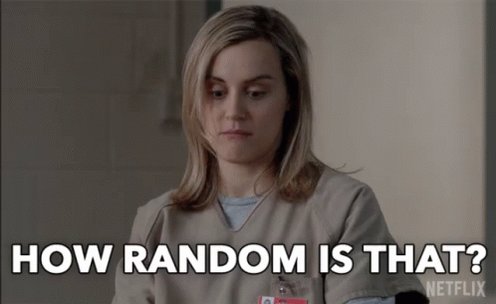
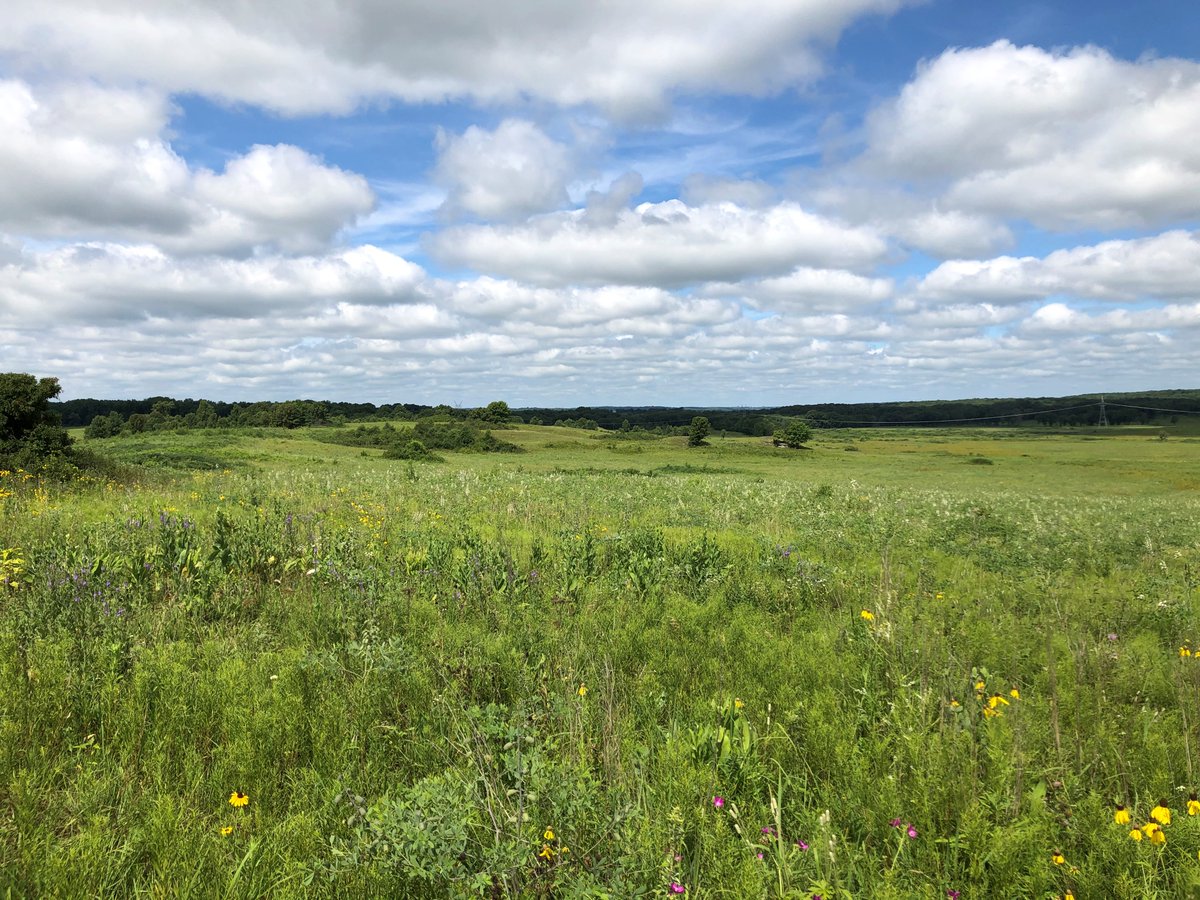
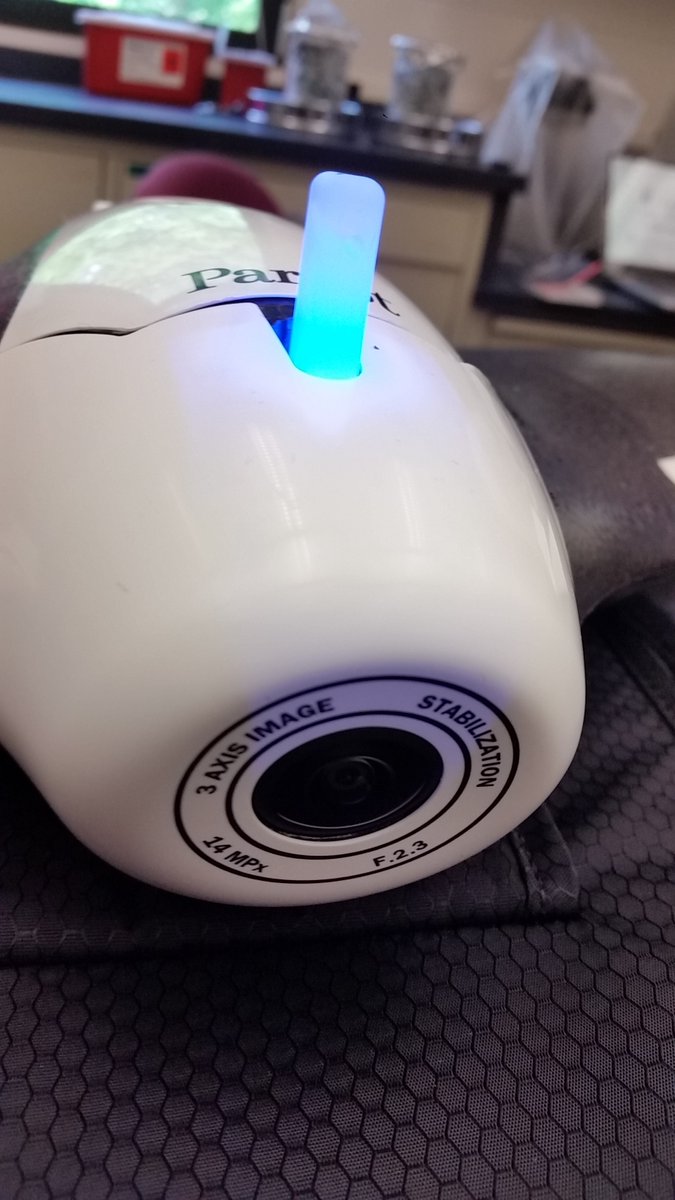
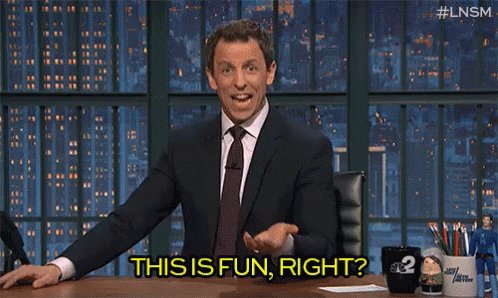
— Ryan C Blackburn (@Blackburn_RC) January 8, 2021
More from Tech
These past few days I've been experimenting with something new that I want to use by myself.
Interestingly, this thread below has been written by that.
Let me show you how it looks like. 👇🏻
When you see localhost up there, you should know that it's truly an experiment! 😀
It's a dead-simple thread writer that will post a series of tweets a.k.a tweetstorm. ⚡️
I've been personally wanting it myself since few months ago, but neglected it intentionally to make sure it's something that I genuinely need.
So why is that important for me? 🙂
I've been a believer of a story. I tell stories all the time, whether it's in the real world or online like this. Our society has moved by that.
If you're interested by stories that move us, read Sapiens!
One of the stories that I've told was from the launch of Poster.
It's been launched multiple times this year, and Twitter has been my go-to place to tell the world about that.
Here comes my frustration.. 😤
Interestingly, this thread below has been written by that.
Let me show you how it looks like. 👇🏻
Recently I just refunded all Poster's sales from Gumroad. Being that said, I decided to not using that service anymore.
— Wilbert Liu \U0001f468\U0001f3fb\u200d\U0001f3a8 (@wilbertliu) November 19, 2018
Here's a little story \U0001f447\U0001f3fb
When you see localhost up there, you should know that it's truly an experiment! 😀
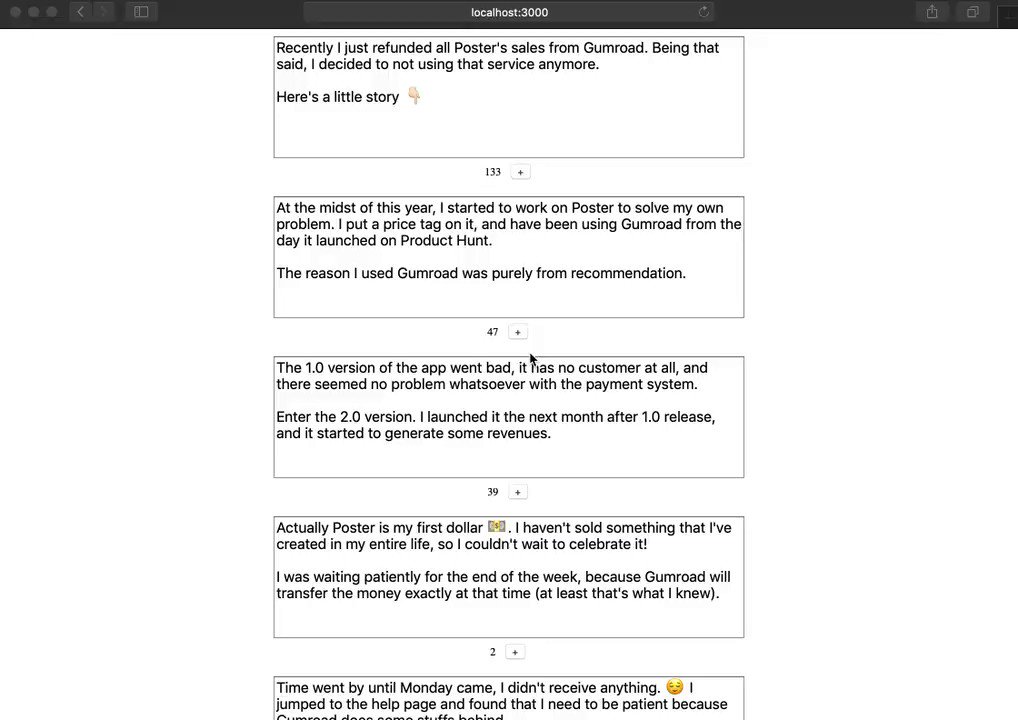
It's a dead-simple thread writer that will post a series of tweets a.k.a tweetstorm. ⚡️
I've been personally wanting it myself since few months ago, but neglected it intentionally to make sure it's something that I genuinely need.
So why is that important for me? 🙂
I've been a believer of a story. I tell stories all the time, whether it's in the real world or online like this. Our society has moved by that.
If you're interested by stories that move us, read Sapiens!
One of the stories that I've told was from the launch of Poster.
It's been launched multiple times this year, and Twitter has been my go-to place to tell the world about that.
Here comes my frustration.. 😤