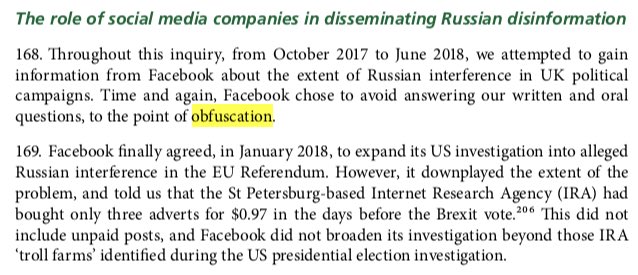
I could create an entire twitter feed of things Facebook has tried to cover up since 2015. Where do you want to start, Mark and Sheryl? https://t.co/1trgupQEH9
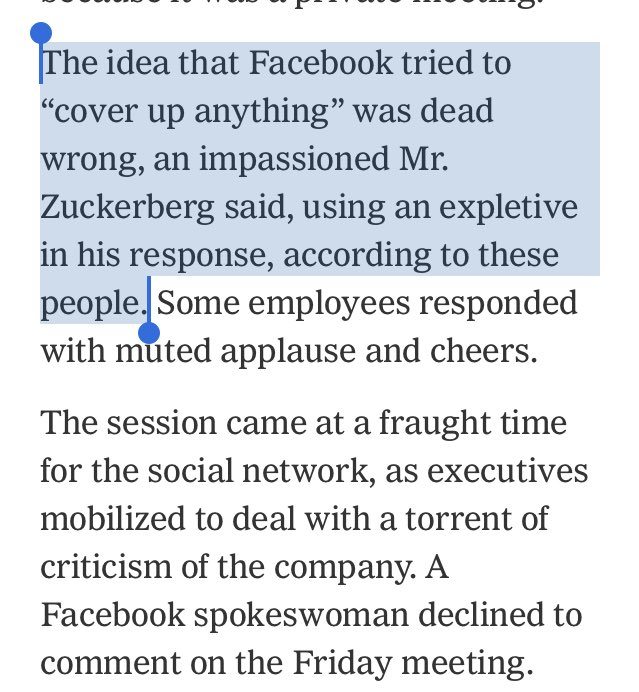
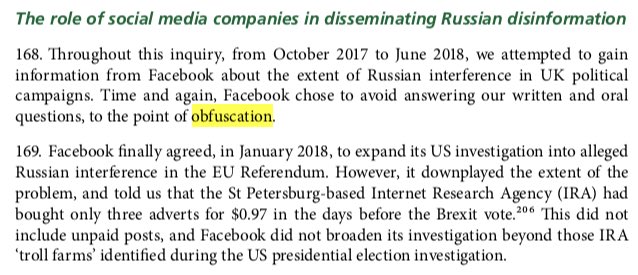
Answer "Facebook has over 30,000 employees. Senior management does not participate in day-today hiring decisions."
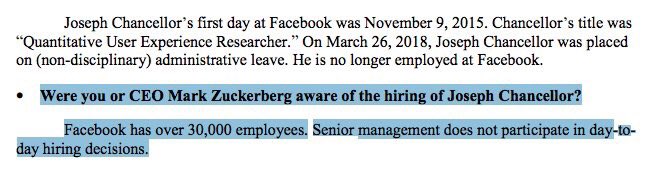
Answer: "He did not become aware of allegations CA may not have deleted data about FB users obtained through Dr. Kogan's app until March of 2018, when
these issues were raised in the media."

A company as powerful as @facebook should be subject to proper scrutiny. Mike Schroepfer, its CTO, told us that the buck stops with Mark Zuckerberg on the Cambridge Analytica scandal, which is why he should come and answer our questions @DamianCollins @IanCLucas pic.twitter.com/0H4VMhtIFu
— Digital, Culture, Media and Sport Committee (@CommonsCMS) May 23, 2018
More from Tech
A brief analysis and comparison of the CSS for Twitter's PWA vs Twitter's legacy desktop website. The difference is dramatic and I'll touch on some reasons why.
Legacy site *downloads* ~630 KB CSS per theme and writing direction.
6,769 rules
9,252 selectors
16.7k declarations
3,370 unique declarations
44 media queries
36 unique colors
50 unique background colors
46 unique font sizes
39 unique z-indices
https://t.co/qyl4Bt1i5x
PWA *incrementally generates* ~30 KB CSS that handles all themes and writing directions.
735 rules
740 selectors
757 declarations
730 unique declarations
0 media queries
11 unique colors
32 unique background colors
15 unique font sizes
7 unique z-indices
https://t.co/w7oNG5KUkJ
The legacy site's CSS is what happens when hundreds of people directly write CSS over many years. Specificity wars, redundancy, a house of cards that can't be fixed. The result is extremely inefficient and error-prone styling that punishes users and developers.
The PWA's CSS is generated on-demand by a JS framework that manages styles and outputs "atomic CSS". The framework can enforce strict constraints and perform optimisations, which is why the CSS is so much smaller and safer. Style conflicts and unbounded CSS growth are avoided.
Legacy site *downloads* ~630 KB CSS per theme and writing direction.
6,769 rules
9,252 selectors
16.7k declarations
3,370 unique declarations
44 media queries
36 unique colors
50 unique background colors
46 unique font sizes
39 unique z-indices
https://t.co/qyl4Bt1i5x

PWA *incrementally generates* ~30 KB CSS that handles all themes and writing directions.
735 rules
740 selectors
757 declarations
730 unique declarations
0 media queries
11 unique colors
32 unique background colors
15 unique font sizes
7 unique z-indices
https://t.co/w7oNG5KUkJ

The legacy site's CSS is what happens when hundreds of people directly write CSS over many years. Specificity wars, redundancy, a house of cards that can't be fixed. The result is extremely inefficient and error-prone styling that punishes users and developers.
The PWA's CSS is generated on-demand by a JS framework that manages styles and outputs "atomic CSS". The framework can enforce strict constraints and perform optimisations, which is why the CSS is so much smaller and safer. Style conflicts and unbounded CSS growth are avoided.
Ok, I’ve told this story a few times, but maybe never here. Here we go. 🧵👇
I was about 6. I was in the car with my mother. We were driving a few hours from home to go to Orlando. My parents were letting me audition for a tv show. It would end up being my first job. I was very excited. But, in the meantime we drove and listened to Rush’s show.
There was some sort of trivia question they posed to the audience. I don’t remember what the riddle was, but I remember I knew the answer right away. It was phrased in this way that was somehow just simpler to see from a kid’s perspective. The answer was CAROUSEL. I was elated.
My mother was THRILLED. She insisted that we call Into the show using her “for emergencies only” giant cell phone. It was this phone:
I called in. The phone rang for a while, but someone answered. It was an impatient-sounding dude. The screener. I said I had the trivia answer. He wasn’t charmed, I could hear him rolling his eyes. He asked me what it was. I told him. “Please hold.”
Wish I had the audio of Rush Limbaugh telling me off on the phone on his show when I was six. In the meantime, RIP.
— Shannon Woodward (@shannonwoodward) February 17, 2021
I was about 6. I was in the car with my mother. We were driving a few hours from home to go to Orlando. My parents were letting me audition for a tv show. It would end up being my first job. I was very excited. But, in the meantime we drove and listened to Rush’s show.
There was some sort of trivia question they posed to the audience. I don’t remember what the riddle was, but I remember I knew the answer right away. It was phrased in this way that was somehow just simpler to see from a kid’s perspective. The answer was CAROUSEL. I was elated.
My mother was THRILLED. She insisted that we call Into the show using her “for emergencies only” giant cell phone. It was this phone:
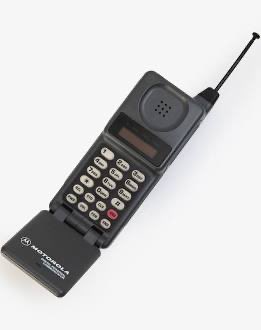
I called in. The phone rang for a while, but someone answered. It was an impatient-sounding dude. The screener. I said I had the trivia answer. He wasn’t charmed, I could hear him rolling his eyes. He asked me what it was. I told him. “Please hold.”
You May Also Like
fascinated by this man, mario cortellucci, and his outsized influence on ontario and GTA politics. cortellucci, who lives in vaughan and ran as a far-right candidate for the italian senate back in 2018 - is a major ford donor...
his name might sound familiar because the new cortellucci vaughan hospital at mackenzie health, the one doug ford has been touting lately as a covid-centric facility, is named after him and his family
but his name also pops up in a LOT of other ford projects. for instance - he controls the long term lease on big parts of toronto's portlands... where doug ford once proposed building an nfl stadium and monorail... https://t.co/weOMJ51bVF
cortellucci, who is a developer, also owns a large chunk of the greenbelt. doug ford's desire to develop the greenbelt has been
and late last year he rolled back the mandate of conservation authorities there, prompting the resignations of several members of the greenbelt advisory
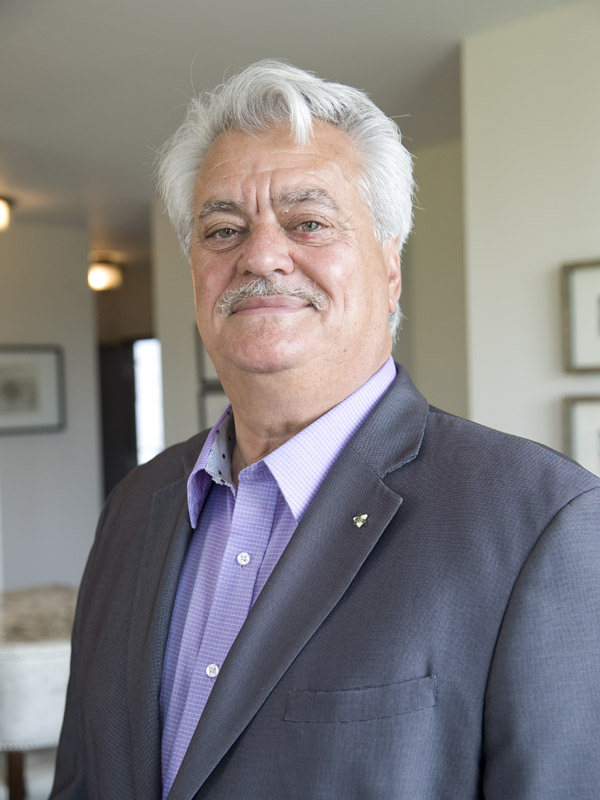
his name might sound familiar because the new cortellucci vaughan hospital at mackenzie health, the one doug ford has been touting lately as a covid-centric facility, is named after him and his family
but his name also pops up in a LOT of other ford projects. for instance - he controls the long term lease on big parts of toronto's portlands... where doug ford once proposed building an nfl stadium and monorail... https://t.co/weOMJ51bVF
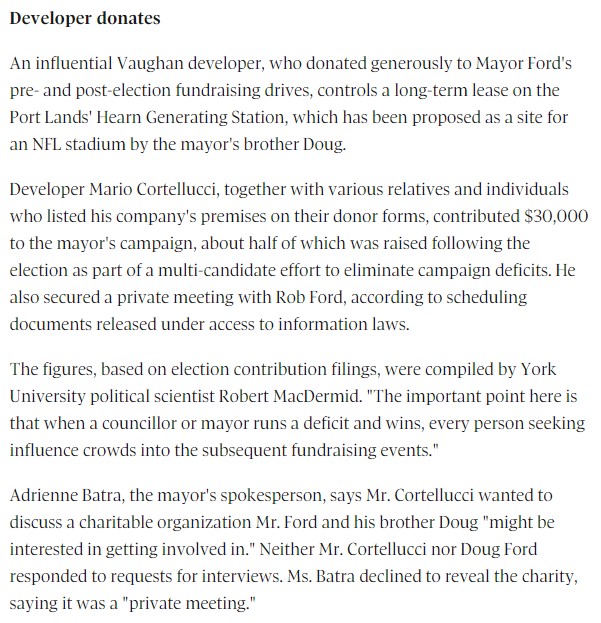
cortellucci, who is a developer, also owns a large chunk of the greenbelt. doug ford's desire to develop the greenbelt has been
and late last year he rolled back the mandate of conservation authorities there, prompting the resignations of several members of the greenbelt advisory