It's 2021! Time for a crash course in four terms that I often see mixed up when people talk about testing: sensitivity, specificity, positive predictive value, negative predictive value.
These terms help us talk about how accurate a test is, but from different viewpoints. 1/
A test that is very *specific* will be very good at accurately ruling out infection in people who are not infected. 3/
Of the 10 people infected, 8 test + (true +), 2 test - (false -).
Of the 90 people uninfected, 89 test - (true -), 1 tests + (false +). 7/
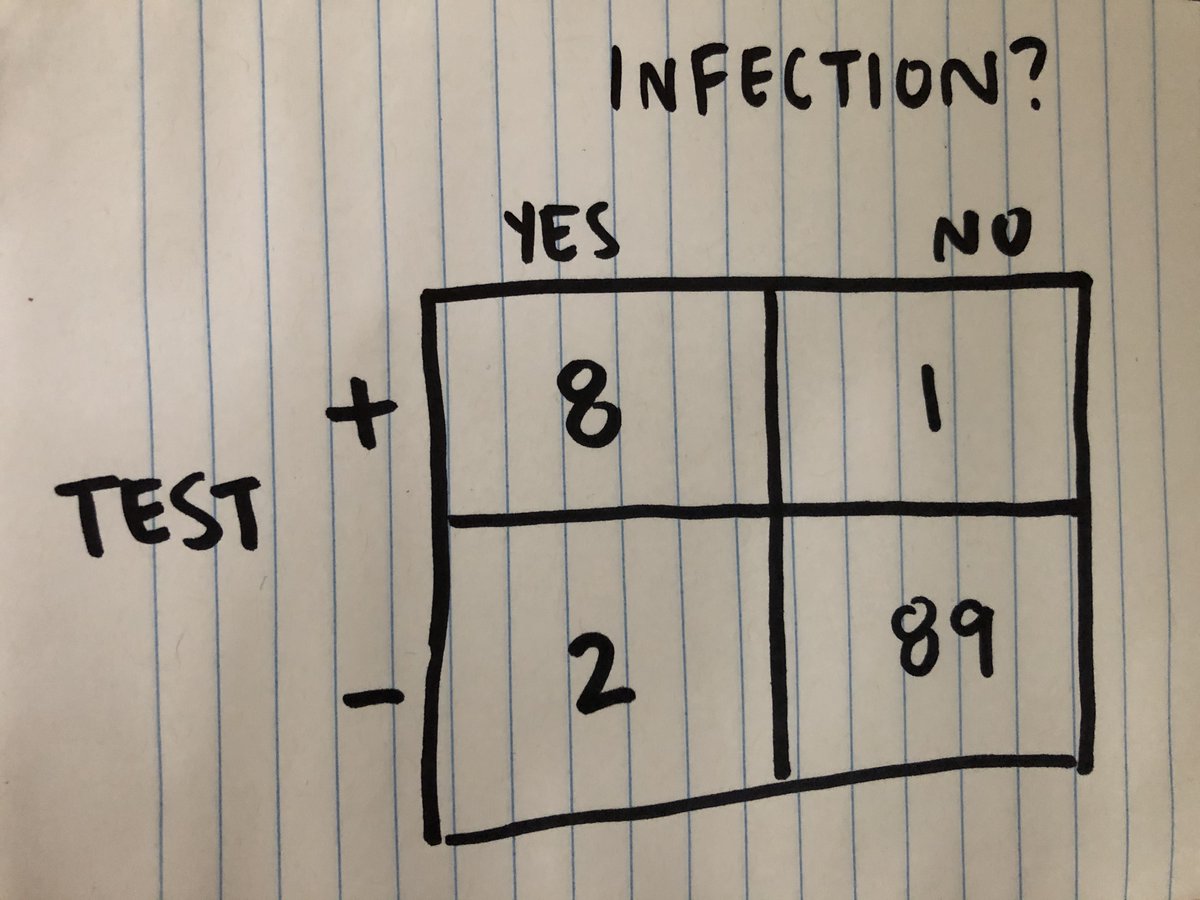
The test's positive predictive value is true positives/(true positives + false positives): 8/9, or 88.9%. It's the proportion of positives, out of all the positives, that were accurate. 10/