1/ I've met so many founders in the last 6 mo who confidentially told me their recent war stories with many top-tier VC firms & their treatment. Two sides to every story but holy moley does it make me sad. I hope the GPs understand that we all talk too. Examples...
More from All
How can we use language supervision to learn better visual representations for robotics?
Introducing Voltron: Language-Driven Representation Learning for Robotics!
Paper: https://t.co/gIsRPtSjKz
Models: https://t.co/NOB3cpATYG
Evaluation: https://t.co/aOzQu95J8z
🧵👇(1 / 12)
Videos of humans performing everyday tasks (Something-Something-v2, Ego4D) offer a rich and diverse resource for learning representations for robotic manipulation.
Yet, an underused part of these datasets are the rich, natural language annotations accompanying each video. (2/12)
The Voltron framework offers a simple way to use language supervision to shape representation learning, building off of prior work in representations for robotics like MVP (https://t.co/Pb0mk9hb4i) and R3M (https://t.co/o2Fkc3fP0e).
The secret is *balance* (3/12)
Starting with a masked autoencoder over frames from these video clips, make a choice:
1) Condition on language and improve our ability to reconstruct the scene.
2) Generate language given the visual representation and improve our ability to describe what's happening. (4/12)
By trading off *conditioning* and *generation* we show that we can learn 1) better representations than prior methods, and 2) explicitly shape the balance of low and high-level features captured.
Why is the ability to shape this balance important? (5/12)
Introducing Voltron: Language-Driven Representation Learning for Robotics!
Paper: https://t.co/gIsRPtSjKz
Models: https://t.co/NOB3cpATYG
Evaluation: https://t.co/aOzQu95J8z
🧵👇(1 / 12)
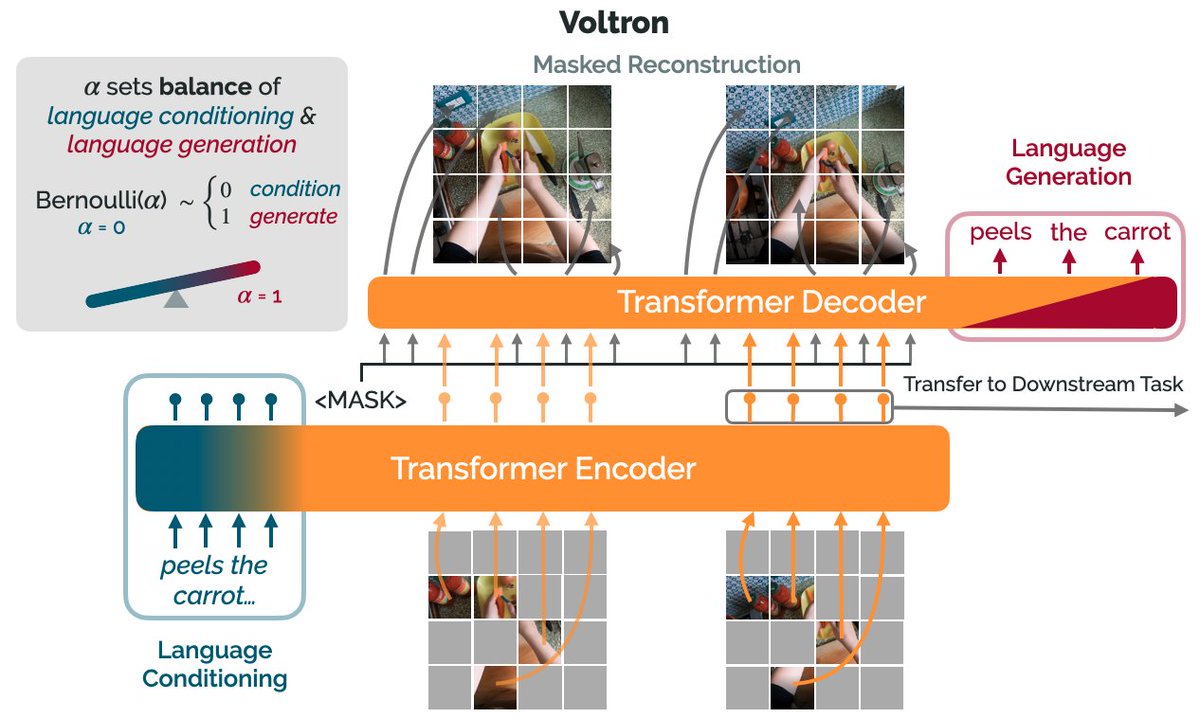
Videos of humans performing everyday tasks (Something-Something-v2, Ego4D) offer a rich and diverse resource for learning representations for robotic manipulation.
Yet, an underused part of these datasets are the rich, natural language annotations accompanying each video. (2/12)
The Voltron framework offers a simple way to use language supervision to shape representation learning, building off of prior work in representations for robotics like MVP (https://t.co/Pb0mk9hb4i) and R3M (https://t.co/o2Fkc3fP0e).
The secret is *balance* (3/12)
Starting with a masked autoencoder over frames from these video clips, make a choice:
1) Condition on language and improve our ability to reconstruct the scene.
2) Generate language given the visual representation and improve our ability to describe what's happening. (4/12)
By trading off *conditioning* and *generation* we show that we can learn 1) better representations than prior methods, and 2) explicitly shape the balance of low and high-level features captured.
Why is the ability to shape this balance important? (5/12)
You May Also Like
Stan Lee’s fictional superheroes lived in the real New York. Here’s where they lived, and why. https://t.co/oV1IGGN8R6
Stan Lee, who died Monday at 95, was born in Manhattan and graduated from DeWitt Clinton High School in the Bronx. His pulp-fiction heroes have come to define much of popular culture in the early 21st century.
Tying Marvel’s stable of pulp-fiction heroes to a real place — New York — served a counterbalance to the sometimes gravity-challenged action and the improbability of the stories. That was just what Stan Lee wanted. https://t.co/rDosqzpP8i
The New York universe hooked readers. And the artists drew what they were familiar with, which made the Marvel universe authentic-looking, down to the water towers atop many of the buildings. https://t.co/rDosqzpP8i
The Avengers Mansion was a Beaux-Arts palace. Fans know it as 890 Fifth Avenue. The Frick Collection, which now occupies the place, uses the address of the front door: 1 East 70th Street.
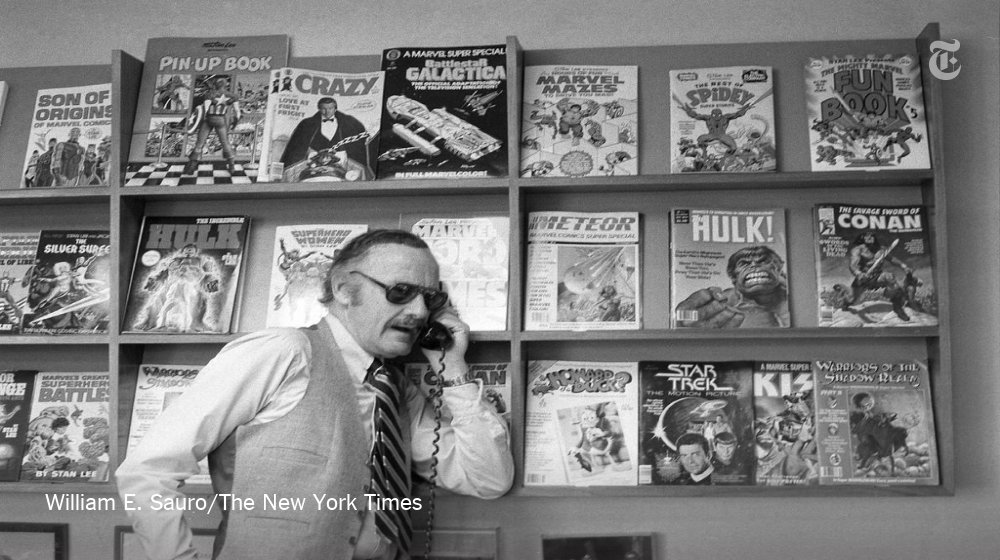
Stan Lee, who died Monday at 95, was born in Manhattan and graduated from DeWitt Clinton High School in the Bronx. His pulp-fiction heroes have come to define much of popular culture in the early 21st century.
Tying Marvel’s stable of pulp-fiction heroes to a real place — New York — served a counterbalance to the sometimes gravity-challenged action and the improbability of the stories. That was just what Stan Lee wanted. https://t.co/rDosqzpP8i
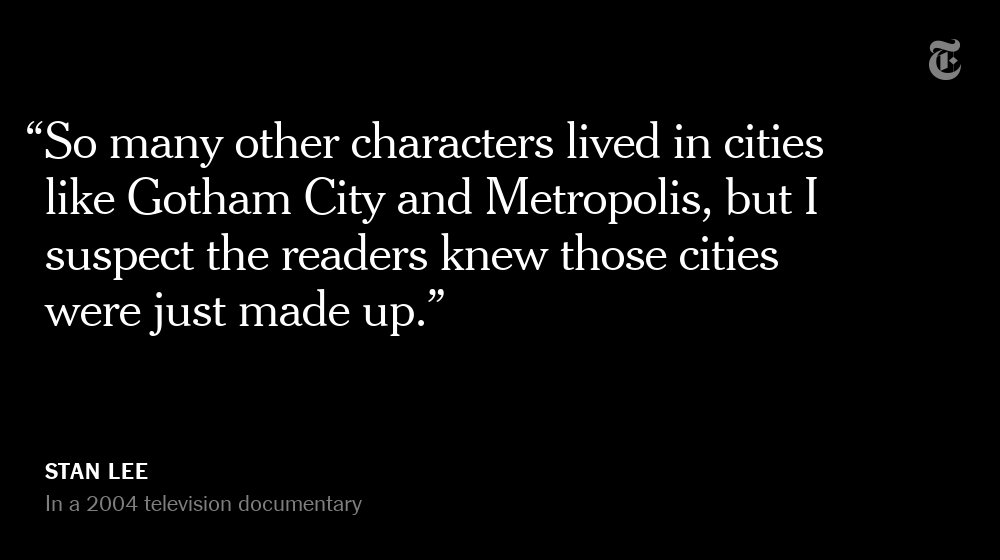
The New York universe hooked readers. And the artists drew what they were familiar with, which made the Marvel universe authentic-looking, down to the water towers atop many of the buildings. https://t.co/rDosqzpP8i
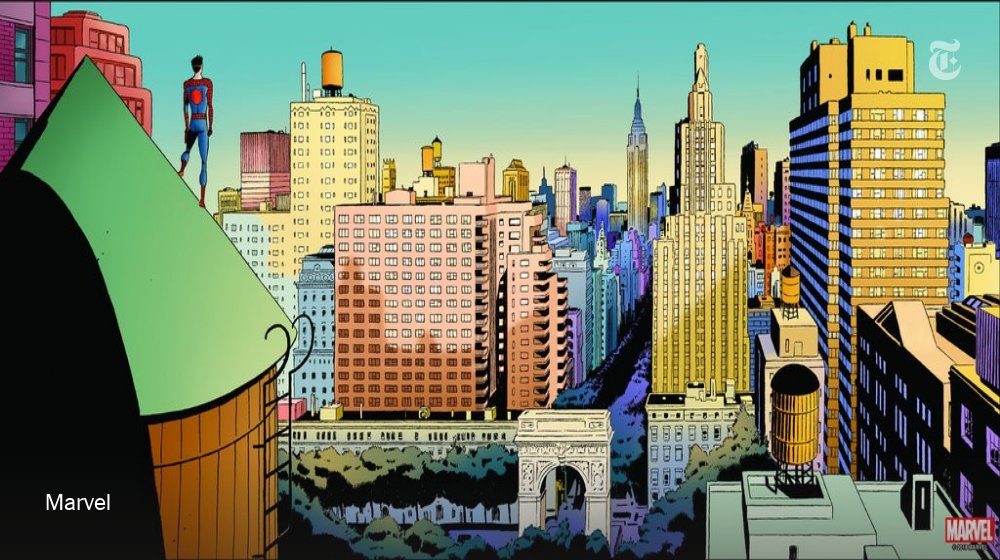
The Avengers Mansion was a Beaux-Arts palace. Fans know it as 890 Fifth Avenue. The Frick Collection, which now occupies the place, uses the address of the front door: 1 East 70th Street.