Once you move above that mark, it becomes exponentially, far and away beyond anything I dreamed, more difficult.
I think about this a lot, both in IT and civil infrastructure. It looks so trivial to “fix” from the outside. In fact, it is incredibly draining to do the entirely crushing work of real policy changes internally. It’s harder than drafting a blank page of how the world should be.
The tragedy of revolutionaries is they design a utopia by a river but discover the impure city they razed was on stilts for a reason.
— SwiftOnSecurity (@SwiftOnSecurity) June 19, 2016
Once you move above that mark, it becomes exponentially, far and away beyond anything I dreamed, more difficult.
God I wish I lived in that world of triviality. In moments, I find myself regretting leaving that place of self-directed autonomy.
More from Tech
You May Also Like
fascinated by this man, mario cortellucci, and his outsized influence on ontario and GTA politics. cortellucci, who lives in vaughan and ran as a far-right candidate for the italian senate back in 2018 - is a major ford donor...
his name might sound familiar because the new cortellucci vaughan hospital at mackenzie health, the one doug ford has been touting lately as a covid-centric facility, is named after him and his family
but his name also pops up in a LOT of other ford projects. for instance - he controls the long term lease on big parts of toronto's portlands... where doug ford once proposed building an nfl stadium and monorail... https://t.co/weOMJ51bVF
cortellucci, who is a developer, also owns a large chunk of the greenbelt. doug ford's desire to develop the greenbelt has been
and late last year he rolled back the mandate of conservation authorities there, prompting the resignations of several members of the greenbelt advisory
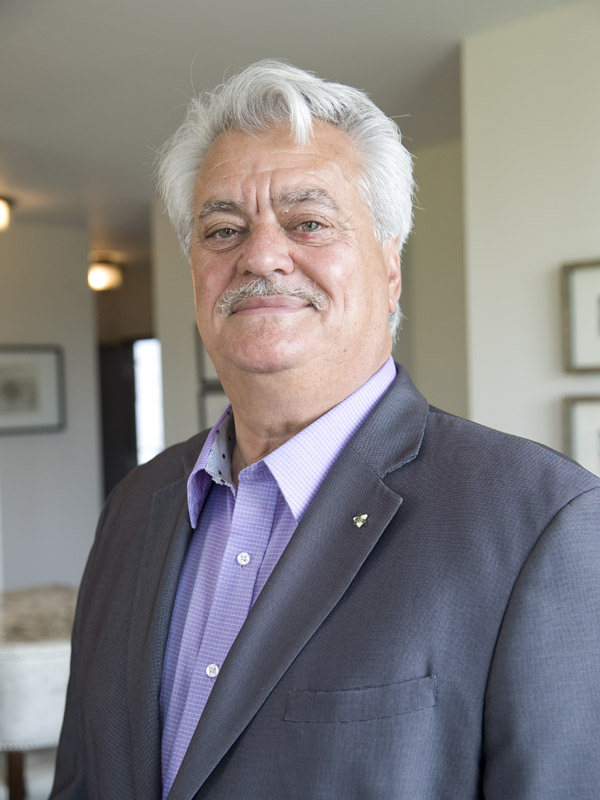
his name might sound familiar because the new cortellucci vaughan hospital at mackenzie health, the one doug ford has been touting lately as a covid-centric facility, is named after him and his family
but his name also pops up in a LOT of other ford projects. for instance - he controls the long term lease on big parts of toronto's portlands... where doug ford once proposed building an nfl stadium and monorail... https://t.co/weOMJ51bVF
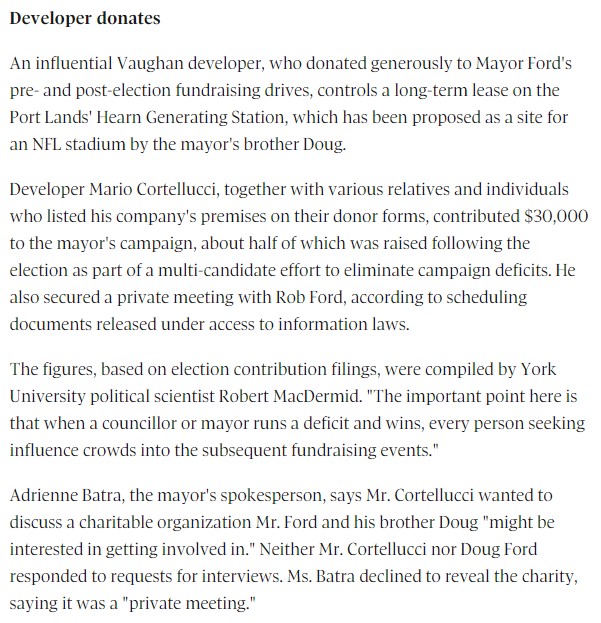
cortellucci, who is a developer, also owns a large chunk of the greenbelt. doug ford's desire to develop the greenbelt has been
and late last year he rolled back the mandate of conservation authorities there, prompting the resignations of several members of the greenbelt advisory