Okay, here we go.
Neural Volume Rendering for Dynamic Scenes
NeRF has shown incredible view synthesis results, but it requires multi-view captures for STATIC scenes.
How can we achieve view synthesis for DYNAMIC scenes from a single video? Here is what I learned from several recent efforts.
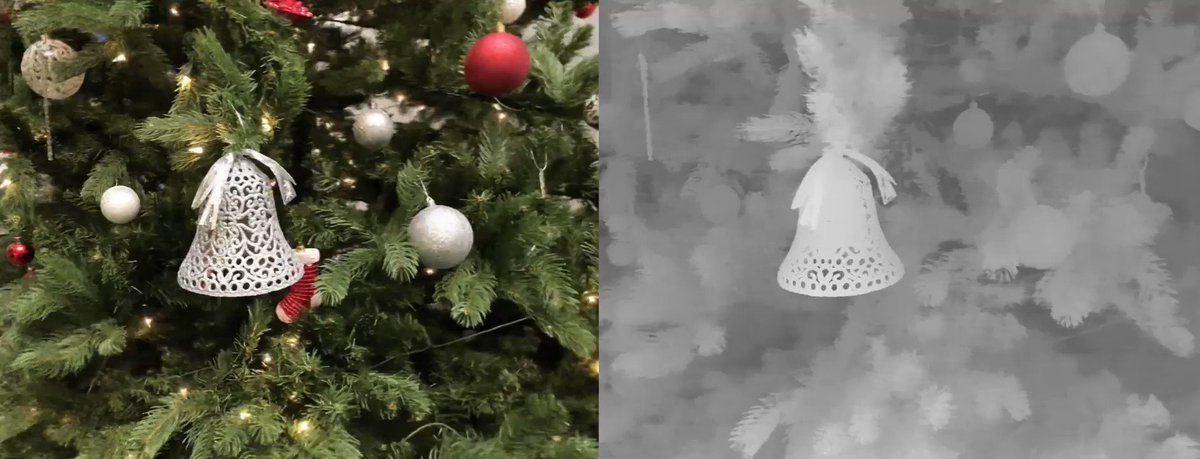
Okay, here we go.
NeRF represents the scene as a 5D continuous volumetric scene function that maps the spatial position and viewing direction to color and density. It then projects the colors/densities to form an image with volume rendering.
Volumetric + Implicit -> Awesome!
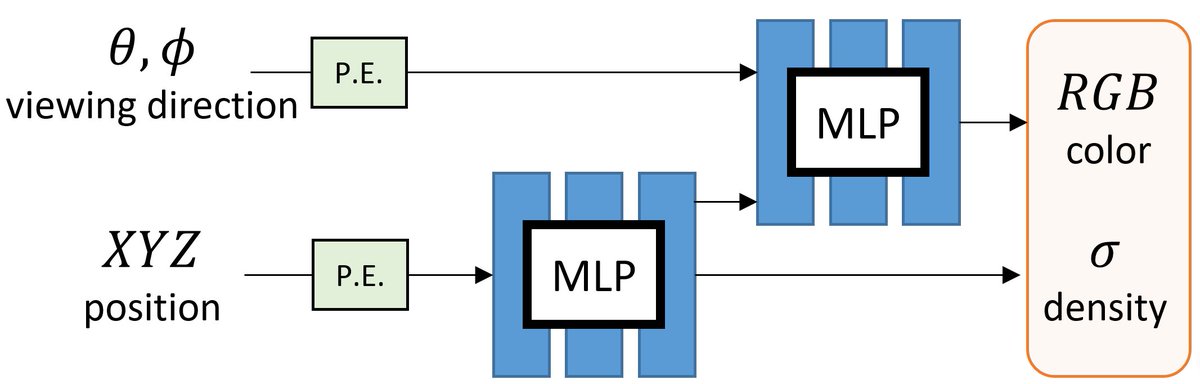
Building on NeRF, one can extend it for handling dynamic scenes with two types of approaches.
A) 4D (or 6D with views) function.
One direct approach is to include TIME as an additional input to learn a DYNAMIC radiance field.
e.g., Video-NeRF, NSFF, NeRFlow
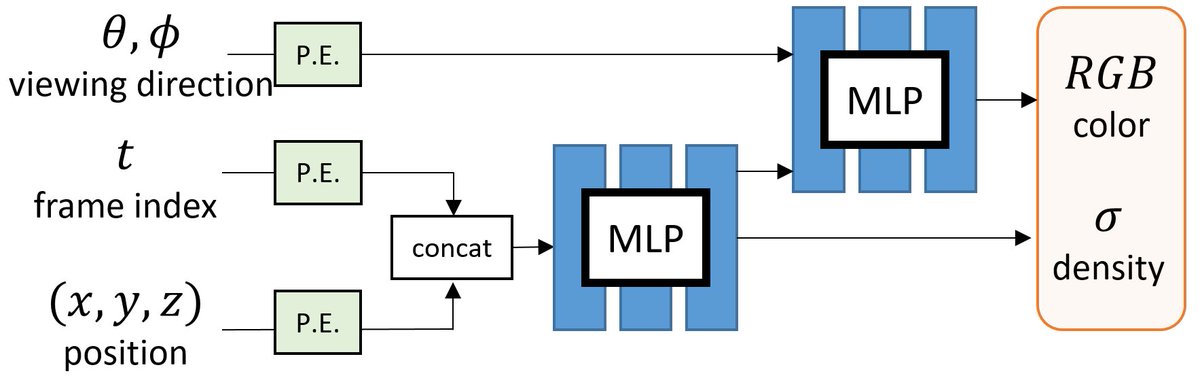
Inspired by non-rigid reconstruction methods, this type of approach learns a radiance field in a canonical frame (template) and predicts deformation for each frame to account for dynamics over time.
e.g., Nerfie, NR-NeRF, D-NeRF
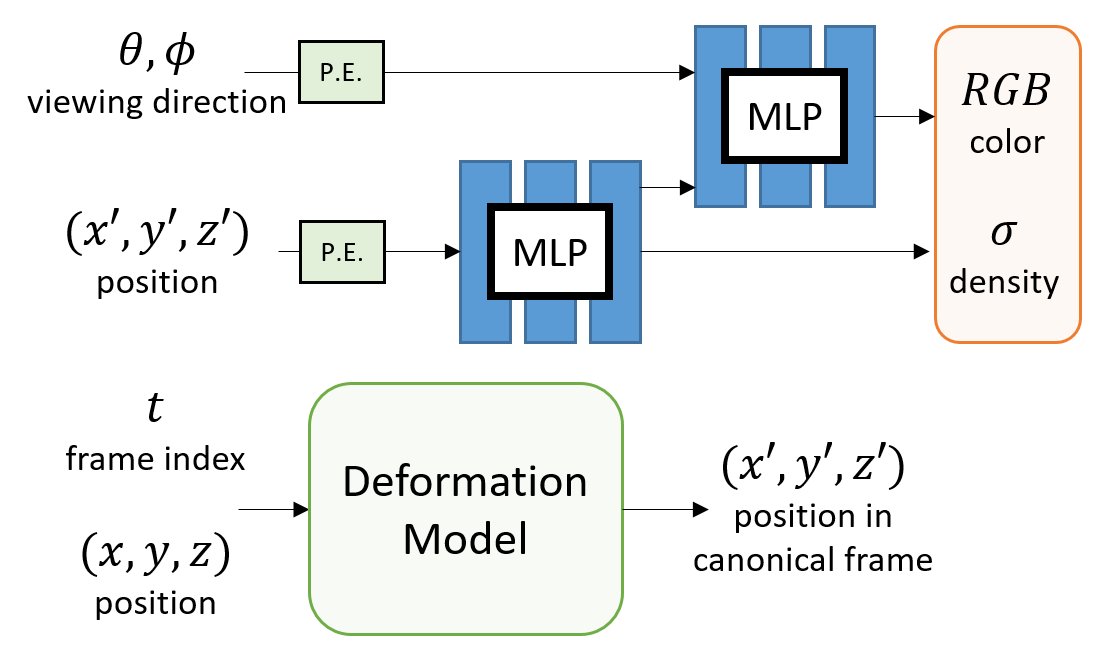
All the methods use an MLP to encode the deformation field. But, how do they differ?
A) INPUT: How to encode the additional time dimension as input?
B) OUTPUT: How to parametrize the deformation field?
One can choose to use EXPLICIT conditioning by treating the frame index t as input.
Alternatively, one can use a learnable LATENT vector for each frame.
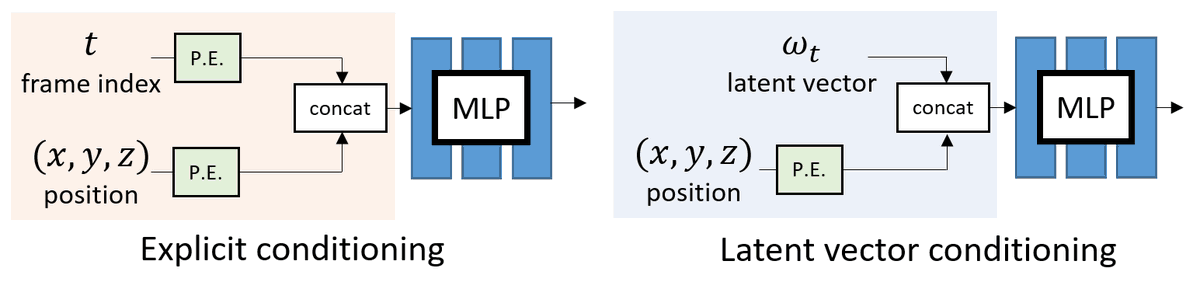
We can either use the MLP to predict
- dense 3D translation vectors (aka scene flow) or
- dense rigid motion field
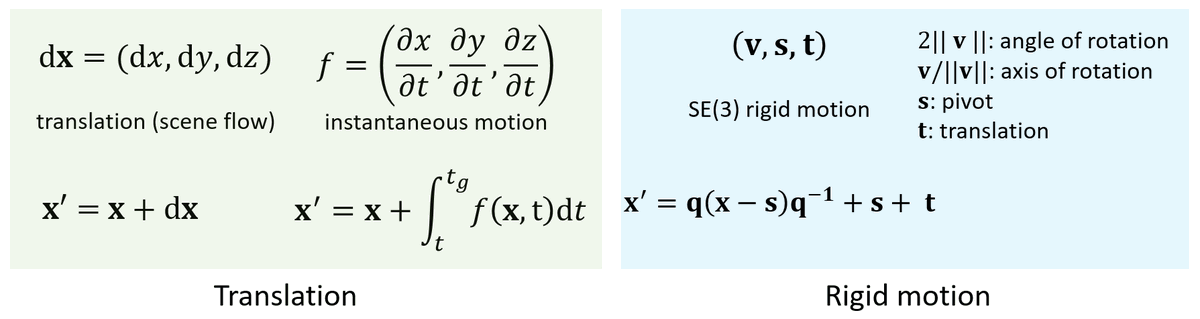
More from Tech
On Wednesday, The New York Times published a blockbuster report on the failures of Facebook’s management team during the past three years. It's.... not flattering, to say the least. Here are six follow-up questions that merit more investigation. 1/
1) During the past year, most of the anger at Facebook has been directed at Mark Zuckerberg. The question now is whether Sheryl Sandberg, the executive charged with solving Facebook’s hardest problems, has caused a few too many of her own. 2/ https://t.co/DTsc3g0hQf
2) One of the juiciest sentences in @nytimes’ piece involves a research group called Definers Public Affairs, which Facebook hired to look into the funding of the company’s opposition. What other tech company was paying Definers to smear Apple? 3/ https://t.co/DTsc3g0hQf
3) The leadership of the Democratic Party has, generally, supported Facebook over the years. But as public opinion turns against the company, prominent Democrats have started to turn, too. What will that relationship look like now? 4/
4) According to the @nytimes, Facebook worked to paint its critics as anti-Semitic, while simultaneously working to spread the idea that George Soros was supporting its critics—a classic tactic of anti-Semitic conspiracy theorists. What exactly were they trying to do there? 5/
1) During the past year, most of the anger at Facebook has been directed at Mark Zuckerberg. The question now is whether Sheryl Sandberg, the executive charged with solving Facebook’s hardest problems, has caused a few too many of her own. 2/ https://t.co/DTsc3g0hQf
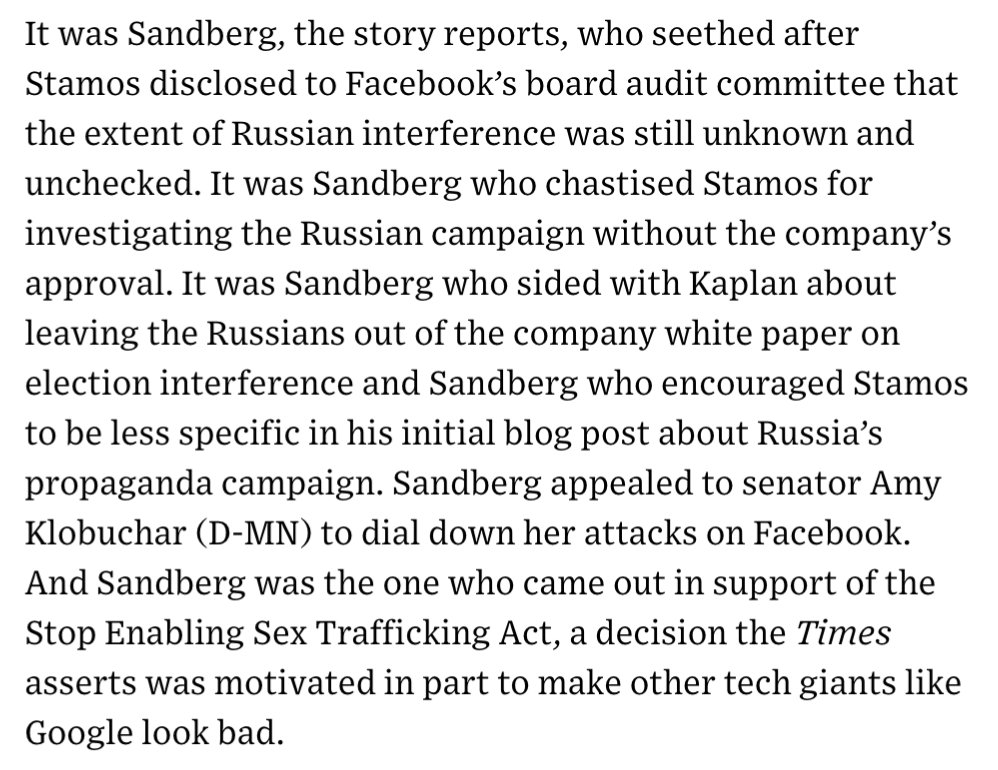
2) One of the juiciest sentences in @nytimes’ piece involves a research group called Definers Public Affairs, which Facebook hired to look into the funding of the company’s opposition. What other tech company was paying Definers to smear Apple? 3/ https://t.co/DTsc3g0hQf
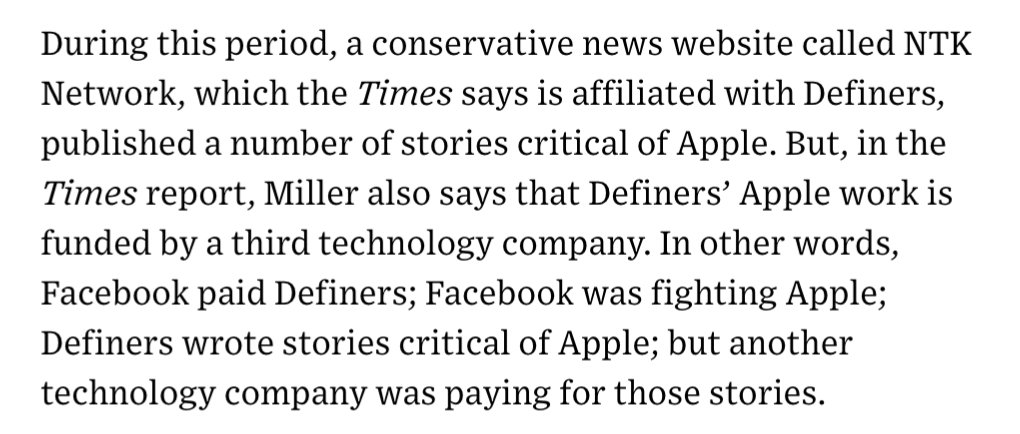
3) The leadership of the Democratic Party has, generally, supported Facebook over the years. But as public opinion turns against the company, prominent Democrats have started to turn, too. What will that relationship look like now? 4/
4) According to the @nytimes, Facebook worked to paint its critics as anti-Semitic, while simultaneously working to spread the idea that George Soros was supporting its critics—a classic tactic of anti-Semitic conspiracy theorists. What exactly were they trying to do there? 5/
After getting good feedback on yesterday's thread on #routemobile I think it is logical to do a bit in-depth technical study. Place #twilio at center, keep #routemobile & #tanla at the periphery & see who is each placed.
This thread is inspired by one of the articles I read on the-ken about #postman API & how they are transforming & expediting software product delivery & consumption, leading to enhanced developer productivity.
We all know that #Twilio offers host of APIs that can be readily used for faster integration by anyone who wants to have communication capabilities. Before we move ahead, let's get a few things cleared out.
Can anyone build the programming capability to process payments or communication capabilities? Yes, but will they, the answer is NO. Companies prefer to consume APIs offered by likes of #Stripe #twilio #Shopify #razorpay etc.
This offers two benefits - faster time to market, of course that means no need to re-invent the wheel + not worrying of compliance around payment process or communication regulations. This makes entire ecosystem extremely agile
So I have been studying this entire communication layer as its relevance is ever growing with more devices coming online, staying connected, and relying on real-time communication. Not that this domain under penetrated, but there is a change underway.
— Ameya (@Finstor85) February 10, 2021
This thread is inspired by one of the articles I read on the-ken about #postman API & how they are transforming & expediting software product delivery & consumption, leading to enhanced developer productivity.
We all know that #Twilio offers host of APIs that can be readily used for faster integration by anyone who wants to have communication capabilities. Before we move ahead, let's get a few things cleared out.
Can anyone build the programming capability to process payments or communication capabilities? Yes, but will they, the answer is NO. Companies prefer to consume APIs offered by likes of #Stripe #twilio #Shopify #razorpay etc.
This offers two benefits - faster time to market, of course that means no need to re-invent the wheel + not worrying of compliance around payment process or communication regulations. This makes entire ecosystem extremely agile
You May Also Like
BREAKING: @CommonsCMS @DamianCollins just released previously sealed #Six4Three @Facebook documents:
Some random interesting tidbits:
1) Zuck approves shutting down platform API access for Twitter's when Vine is released #competition
2) Facebook engineered ways to access user's call history w/o alerting users:
Team considered access to call history considered 'high PR risk' but 'growth team will charge ahead'. @Facebook created upgrade path to access data w/o subjecting users to Android permissions dialogue.
3) The above also confirms @kashhill and other's suspicion that call history was used to improve PYMK (People You May Know) suggestions and newsfeed rankings.
4) Docs also shed more light into @dseetharaman's story on @Facebook monitoring users' @Onavo VPN activity to determine what competitors to mimic or acquire in 2013.
https://t.co/PwiRIL3v9x
Some random interesting tidbits:
1) Zuck approves shutting down platform API access for Twitter's when Vine is released #competition
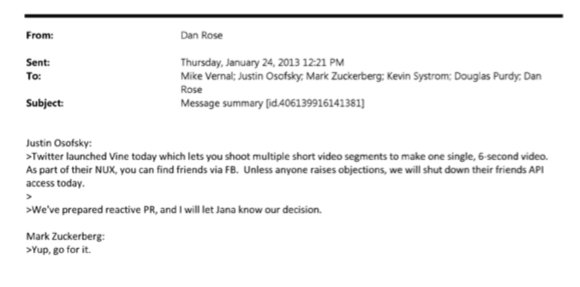
2) Facebook engineered ways to access user's call history w/o alerting users:
Team considered access to call history considered 'high PR risk' but 'growth team will charge ahead'. @Facebook created upgrade path to access data w/o subjecting users to Android permissions dialogue.
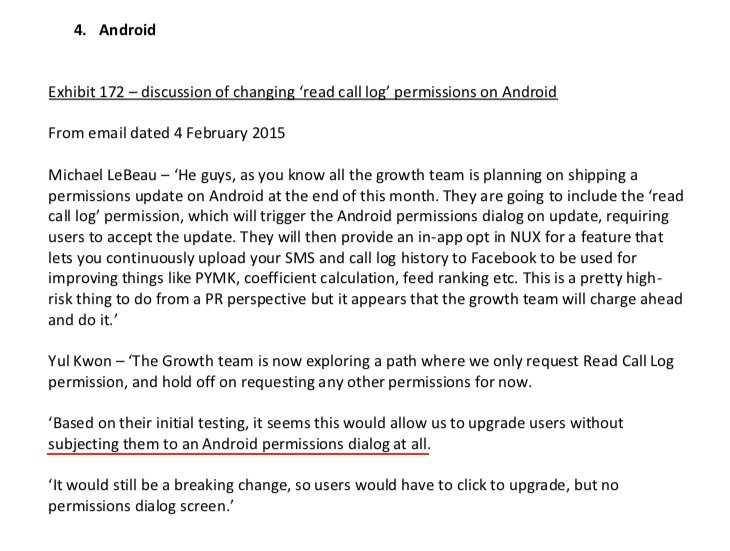
3) The above also confirms @kashhill and other's suspicion that call history was used to improve PYMK (People You May Know) suggestions and newsfeed rankings.
4) Docs also shed more light into @dseetharaman's story on @Facebook monitoring users' @Onavo VPN activity to determine what competitors to mimic or acquire in 2013.
https://t.co/PwiRIL3v9x
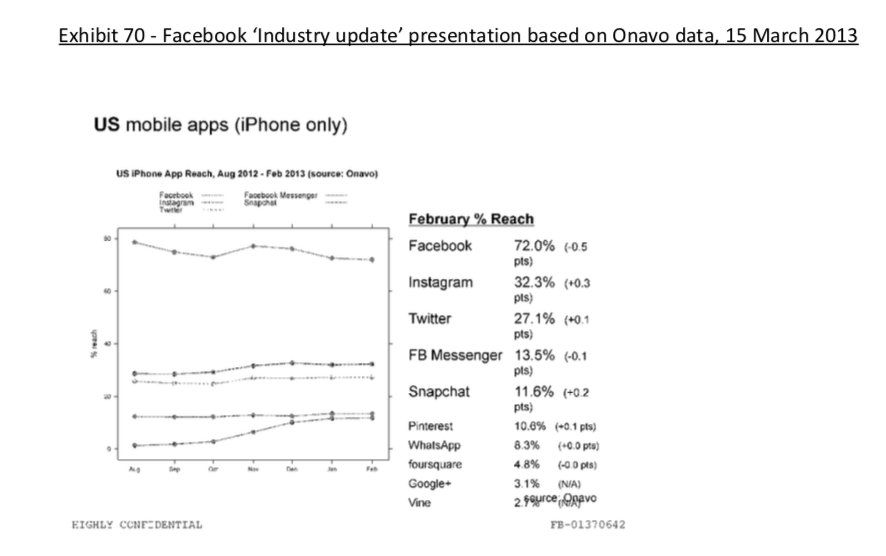