Here's an overview of key adoption metrics for deep learning frameworks over 2020: downloads, developer surveys, job posts, scientific publications, Colab usage, Kaggle notebooks usage, GitHub data.
TensorFlow/Keras = #1 deep learning solution.

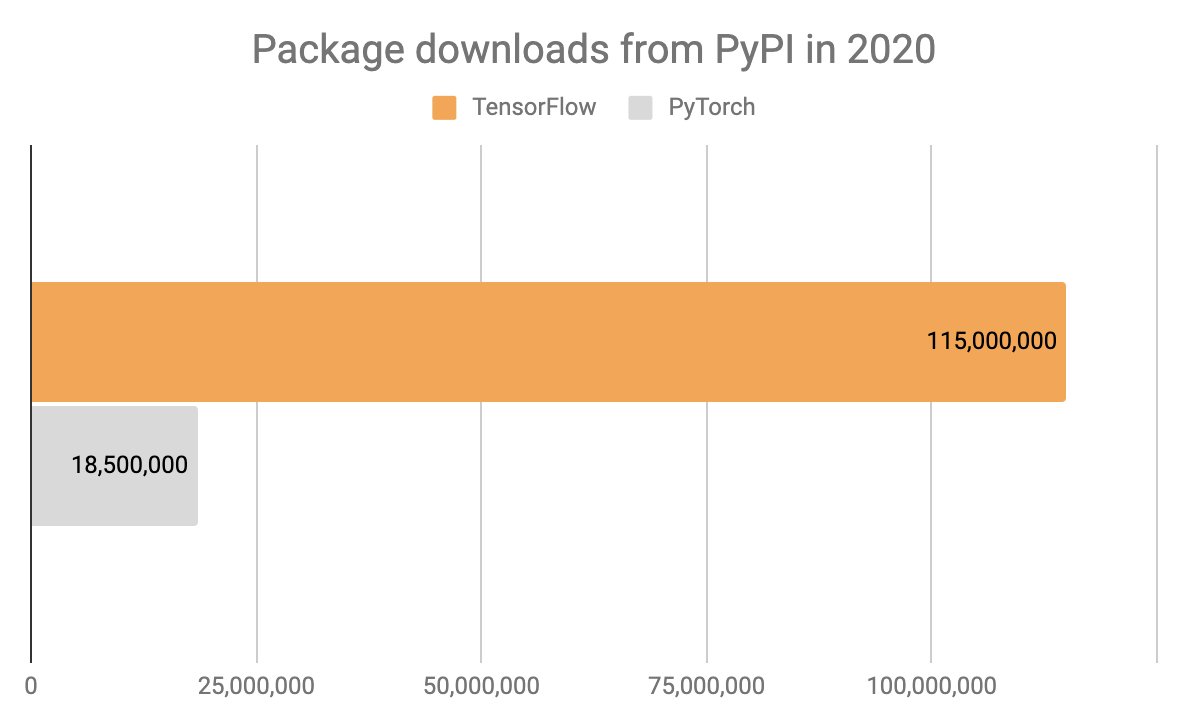
In a way, this metric reflects usage in production.
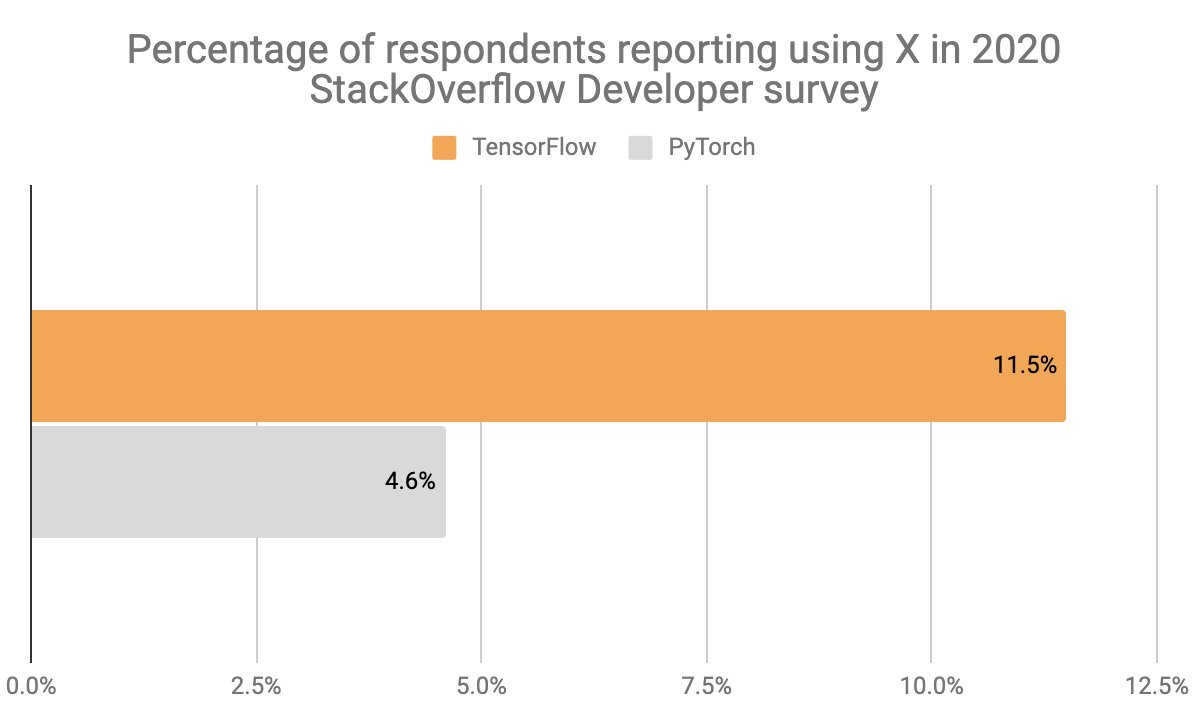
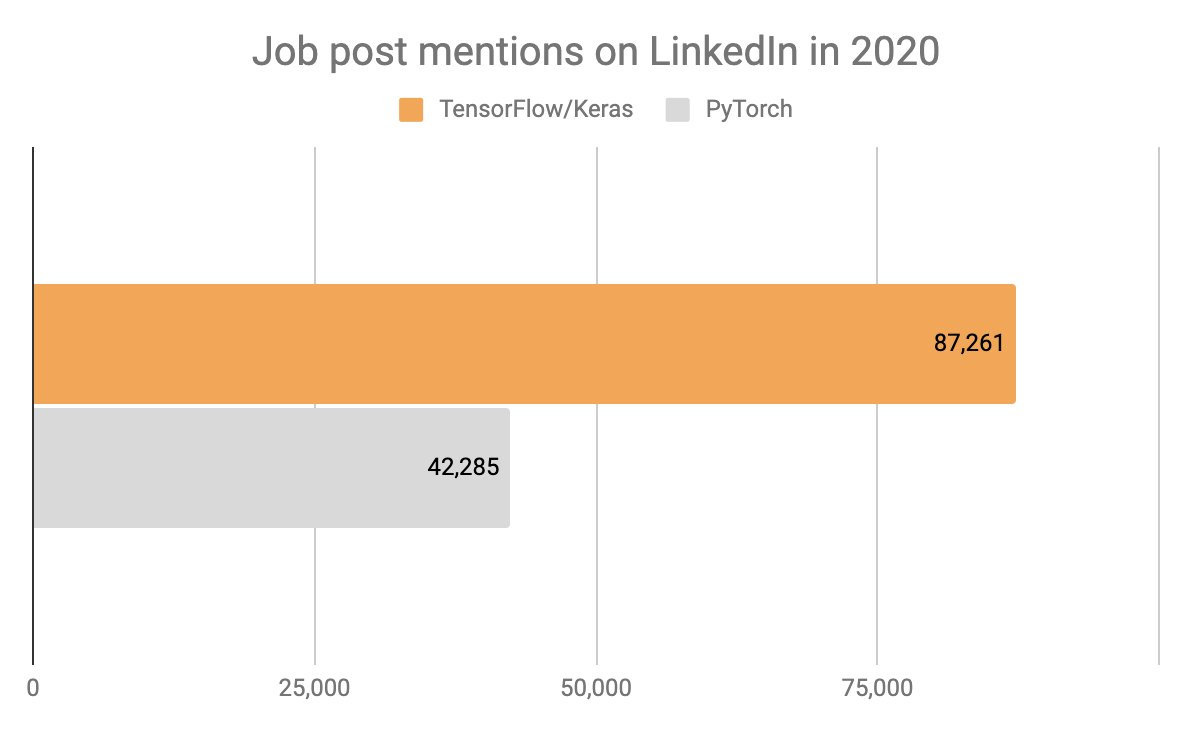
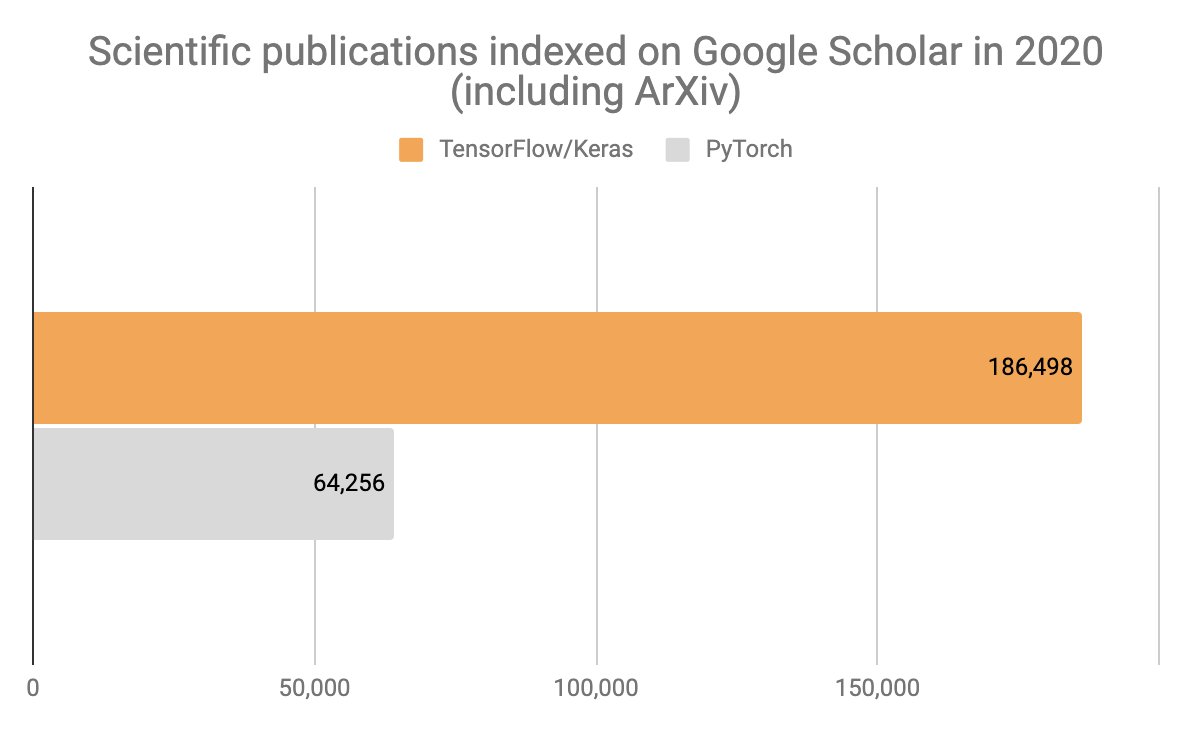
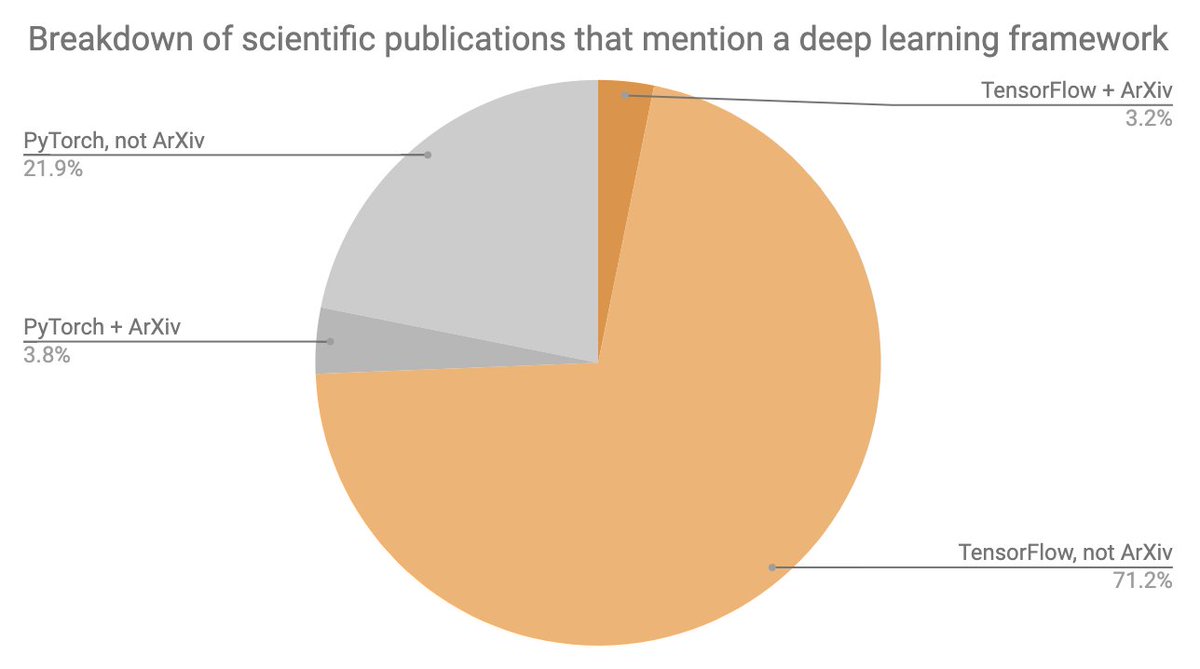
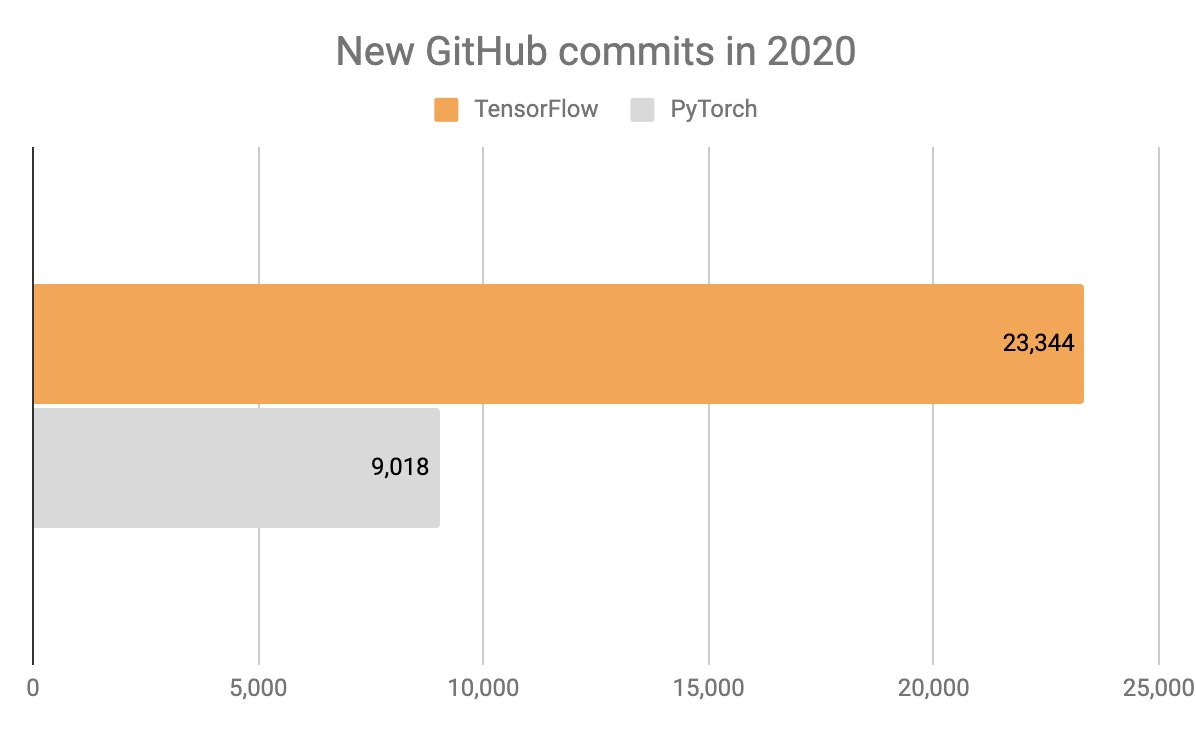
More from Internet
You May Also Like
Krugman is, of course, right about this. BUT, note that universities can do a lot to revitalize declining and rural regions.
See this thing that @lymanstoneky wrote:
And see this thing that I wrote:
And see this book that @JamesFallows wrote:
And see this other thing that I wrote:
One thing I've been noticing about responses to today's column is that many people still don't get how strong the forces behind regional divergence are, and how hard to reverse 1/ https://t.co/Ft2aH1NcQt
— Paul Krugman (@paulkrugman) November 20, 2018
See this thing that @lymanstoneky wrote:
And see this thing that I wrote:
And see this book that @JamesFallows wrote:
And see this other thing that I wrote: