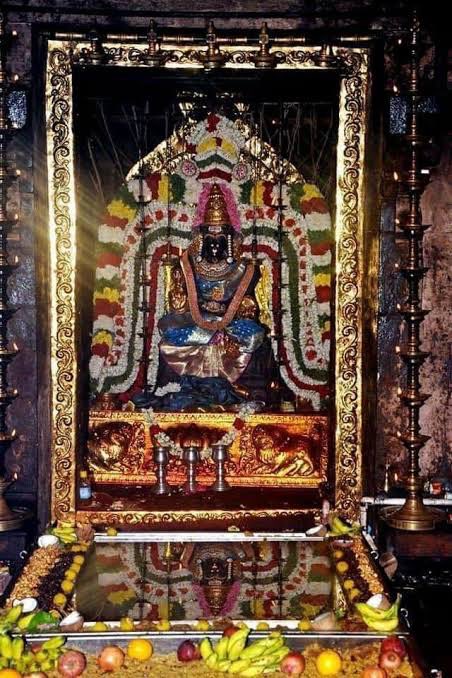
🕉 Centuries old Beautiful Thirumeeyachur Lalithambikai Temple & Lord Mahadev Meghanathaswami ,TN-The Kshetra where Sri Lalitha Sahasranamam -Sanskrit hymn describing the beauty of Goddess in 1000 names by Lord Hayagriva to Sage Agastyar was given as Upadesam 🚩1/5 @twttdc
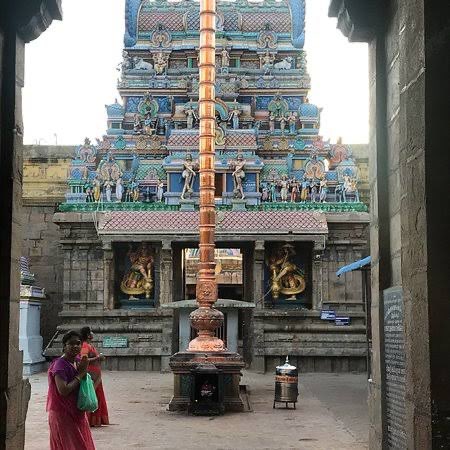
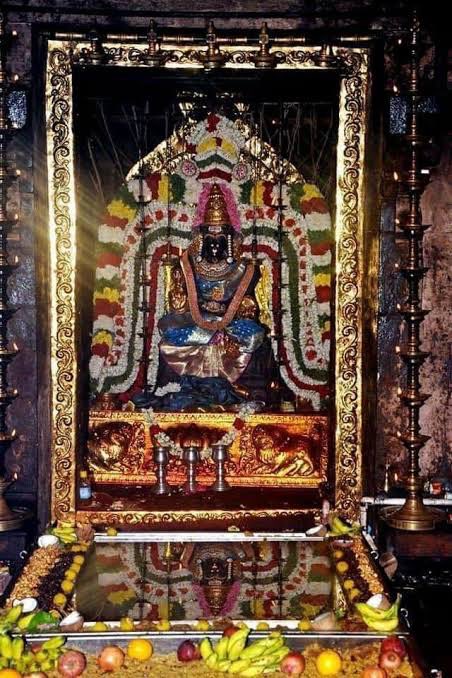
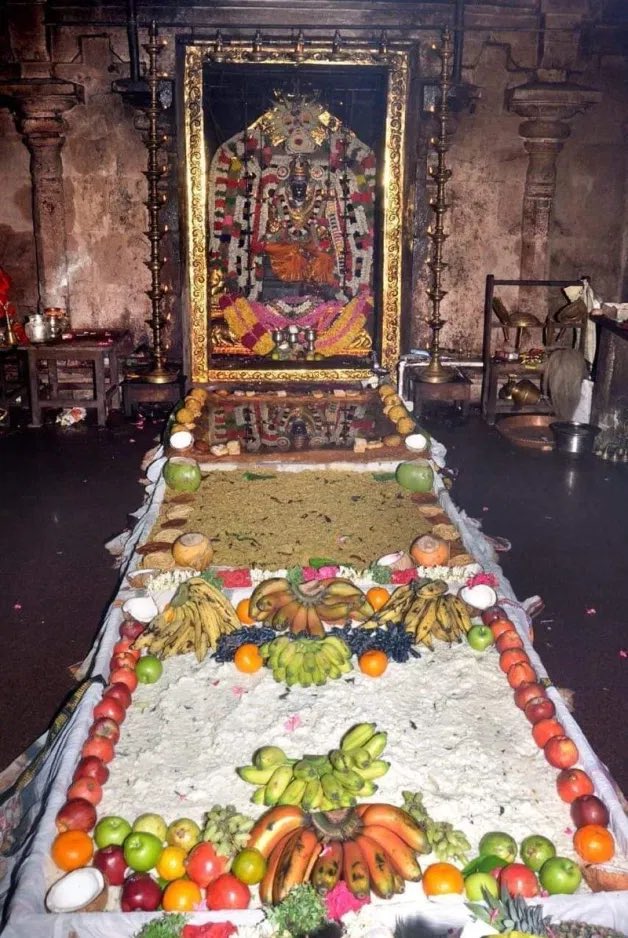
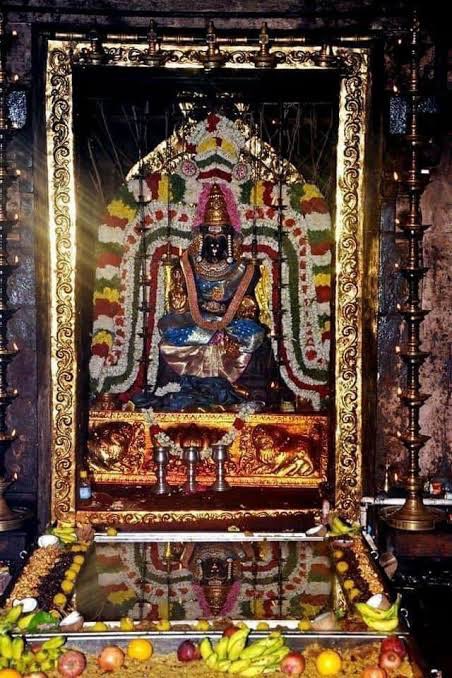
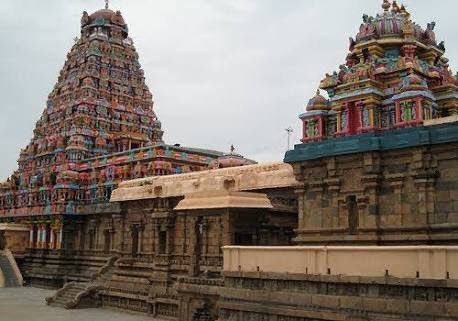
More from All
How can we use language supervision to learn better visual representations for robotics?
Introducing Voltron: Language-Driven Representation Learning for Robotics!
Paper: https://t.co/gIsRPtSjKz
Models: https://t.co/NOB3cpATYG
Evaluation: https://t.co/aOzQu95J8z
🧵👇(1 / 12)
Videos of humans performing everyday tasks (Something-Something-v2, Ego4D) offer a rich and diverse resource for learning representations for robotic manipulation.
Yet, an underused part of these datasets are the rich, natural language annotations accompanying each video. (2/12)
The Voltron framework offers a simple way to use language supervision to shape representation learning, building off of prior work in representations for robotics like MVP (https://t.co/Pb0mk9hb4i) and R3M (https://t.co/o2Fkc3fP0e).
The secret is *balance* (3/12)
Starting with a masked autoencoder over frames from these video clips, make a choice:
1) Condition on language and improve our ability to reconstruct the scene.
2) Generate language given the visual representation and improve our ability to describe what's happening. (4/12)
By trading off *conditioning* and *generation* we show that we can learn 1) better representations than prior methods, and 2) explicitly shape the balance of low and high-level features captured.
Why is the ability to shape this balance important? (5/12)
Introducing Voltron: Language-Driven Representation Learning for Robotics!
Paper: https://t.co/gIsRPtSjKz
Models: https://t.co/NOB3cpATYG
Evaluation: https://t.co/aOzQu95J8z
🧵👇(1 / 12)
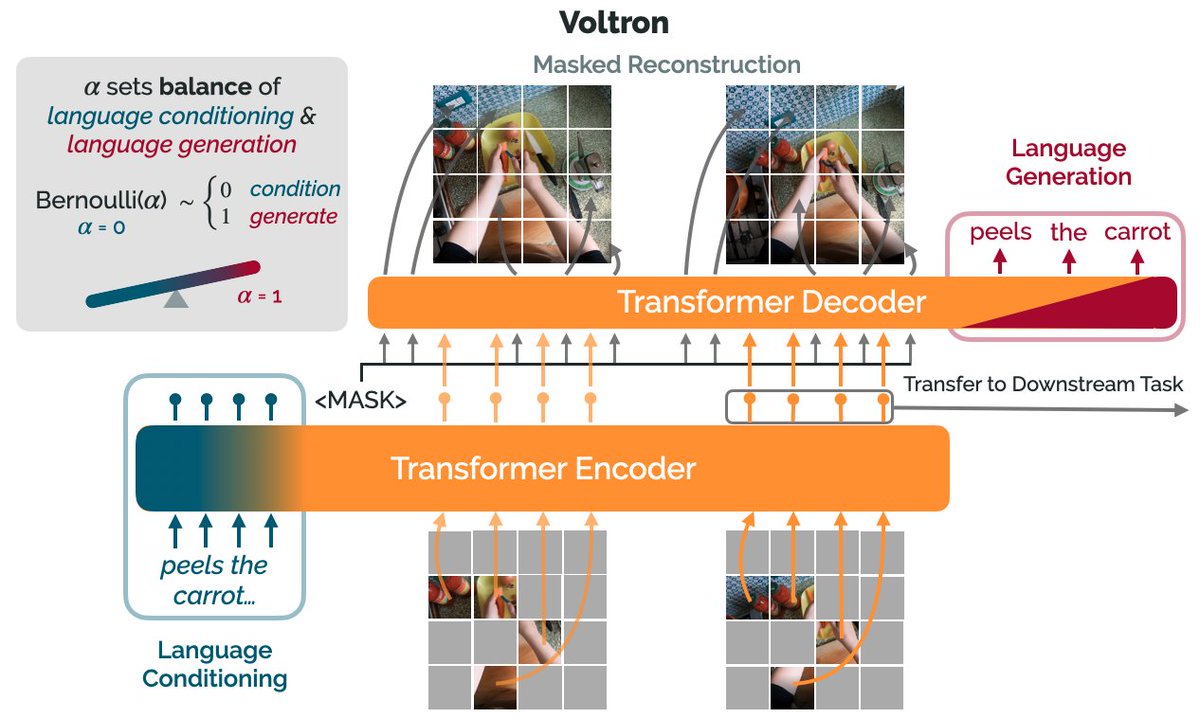
Videos of humans performing everyday tasks (Something-Something-v2, Ego4D) offer a rich and diverse resource for learning representations for robotic manipulation.
Yet, an underused part of these datasets are the rich, natural language annotations accompanying each video. (2/12)
The Voltron framework offers a simple way to use language supervision to shape representation learning, building off of prior work in representations for robotics like MVP (https://t.co/Pb0mk9hb4i) and R3M (https://t.co/o2Fkc3fP0e).
The secret is *balance* (3/12)
Starting with a masked autoencoder over frames from these video clips, make a choice:
1) Condition on language and improve our ability to reconstruct the scene.
2) Generate language given the visual representation and improve our ability to describe what's happening. (4/12)
By trading off *conditioning* and *generation* we show that we can learn 1) better representations than prior methods, and 2) explicitly shape the balance of low and high-level features captured.
Why is the ability to shape this balance important? (5/12)