Categories Google
7 days
30 days
All time
Recent
Popular
Day 14 #31DaysofML
🤔 How to pick the right #GoogleCloud #MachineLearning tool for your application?
Answer these questions
❓ What's your teams ML expertise?
❓ How much control/abstraction do you need?
❓ Would you like to handle the infrastructure components?
🧵 👇
created this pyramid to explain the idea.
As you move up the pyramid, less ML expertise is required, and you also don’t need to worry as much about the infrastructure behind your model.
To lear more watch this video 👉 https://t.co/EqJNDmTfhV
#31DaysofML 2/10
If you’re using Open source ML frameworks (#TensorFlow) to build the models, you get the flexibility of moving your workloads across different development & deployment environments. But, you need to manage all the infrastructure yourself for training & serving
#31DaysofML 3/10
Deep Learning VMs provide managed, click-to-deploy VMs for processing data & training the model
🔹 Popular ML frameworks pre-installed
🔹 Reduces the overhead of managing & allocating compute & storage required
🔹 But you figure out how you’ll serve those models
#31DaysofML 4/10
Kubeflow - OS project for deploying ML workloads on #Kubernetes
🔹 Helps configure a multi-step ML pipeline including pre-processing data, training & serving the model
🔹 Run it on-premise or on any cloud
🔹 You’ll still need to configure where it’s managed
#31DaysofML 5/10
🤔 How to pick the right #GoogleCloud #MachineLearning tool for your application?
Answer these questions
❓ What's your teams ML expertise?
❓ How much control/abstraction do you need?
❓ Would you like to handle the infrastructure components?
🧵 👇
created this pyramid to explain the idea.
As you move up the pyramid, less ML expertise is required, and you also don’t need to worry as much about the infrastructure behind your model.
To lear more watch this video 👉 https://t.co/EqJNDmTfhV
#31DaysofML 2/10
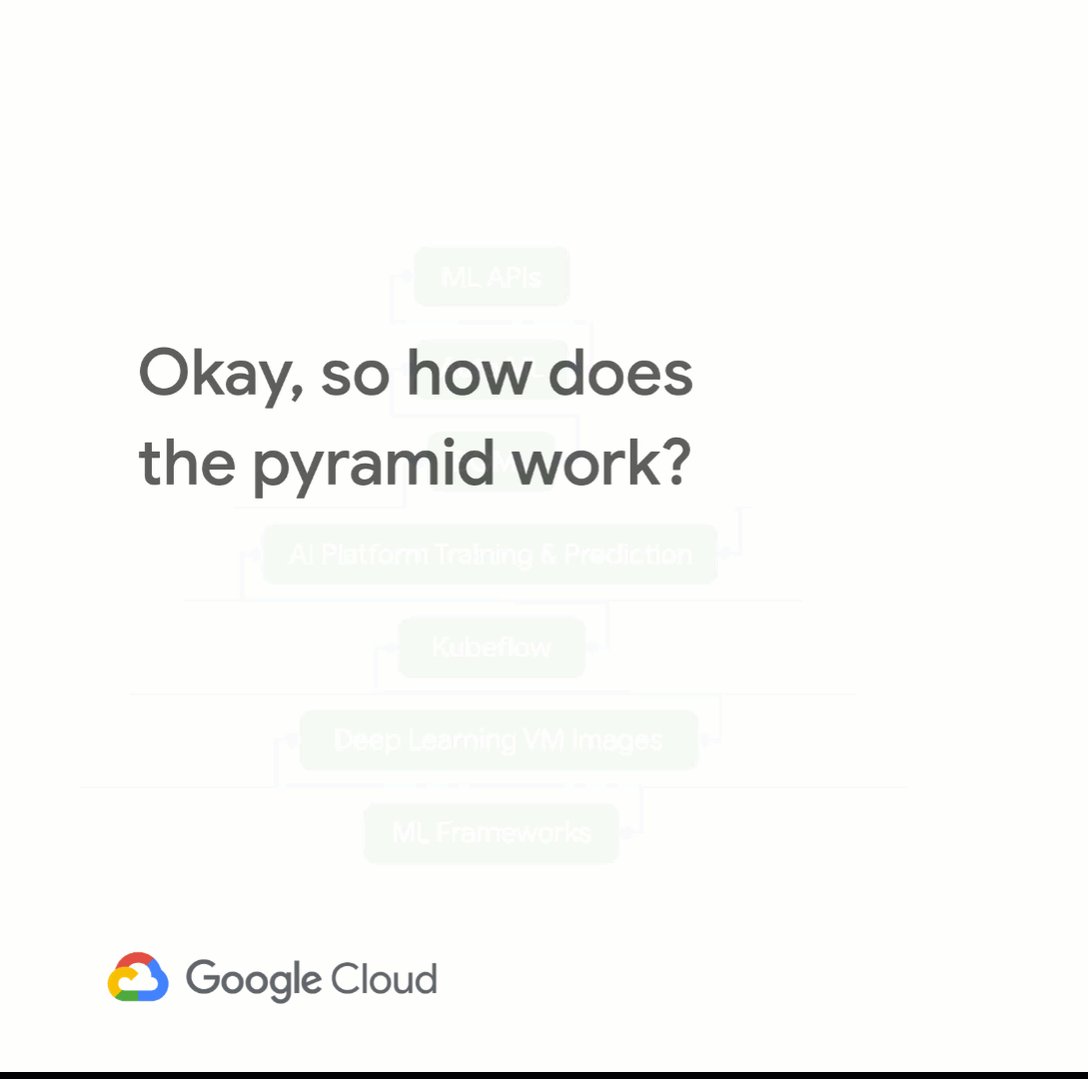
If you’re using Open source ML frameworks (#TensorFlow) to build the models, you get the flexibility of moving your workloads across different development & deployment environments. But, you need to manage all the infrastructure yourself for training & serving
#31DaysofML 3/10
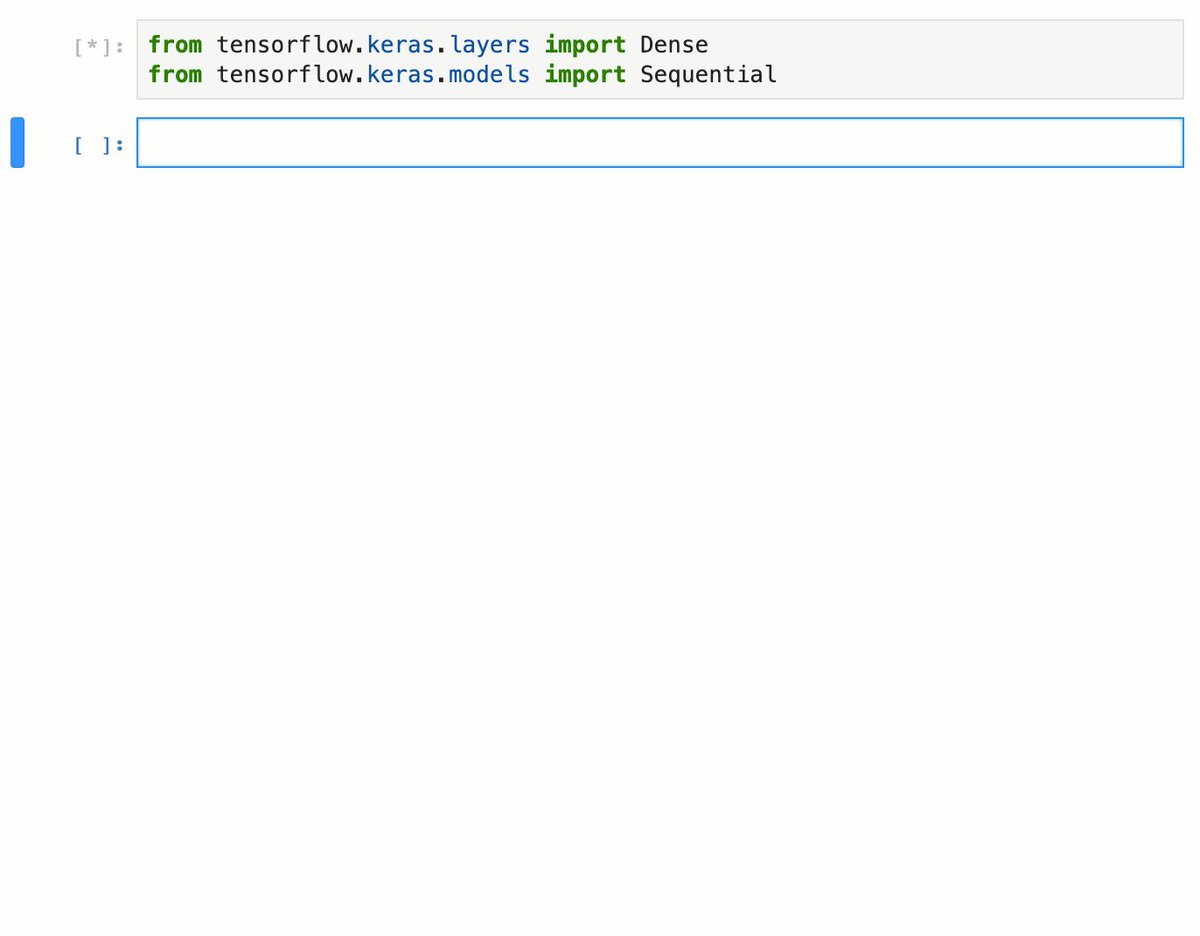
Deep Learning VMs provide managed, click-to-deploy VMs for processing data & training the model
🔹 Popular ML frameworks pre-installed
🔹 Reduces the overhead of managing & allocating compute & storage required
🔹 But you figure out how you’ll serve those models
#31DaysofML 4/10
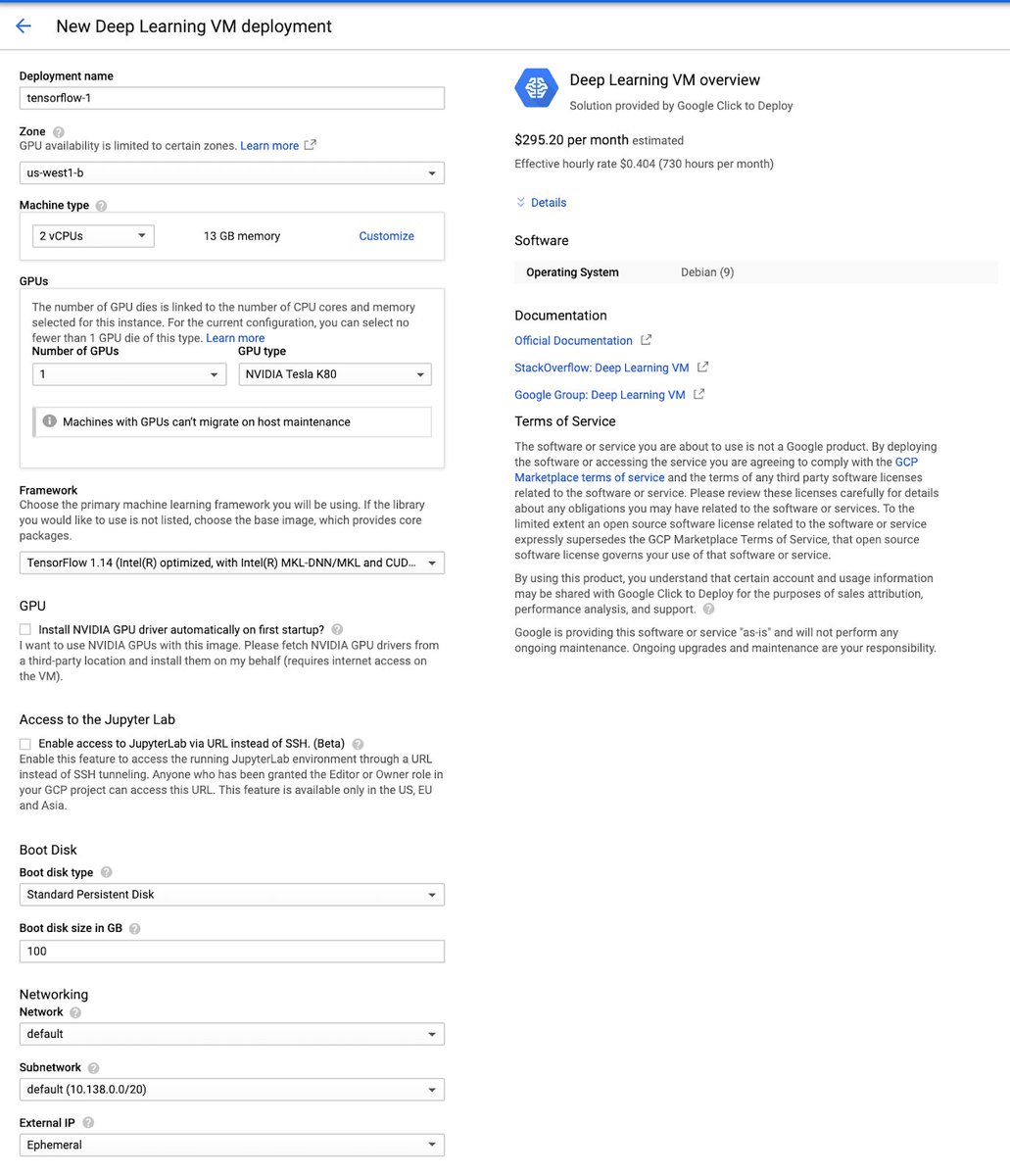
Kubeflow - OS project for deploying ML workloads on #Kubernetes
🔹 Helps configure a multi-step ML pipeline including pre-processing data, training & serving the model
🔹 Run it on-premise or on any cloud
🔹 You’ll still need to configure where it’s managed
#31DaysofML 5/10
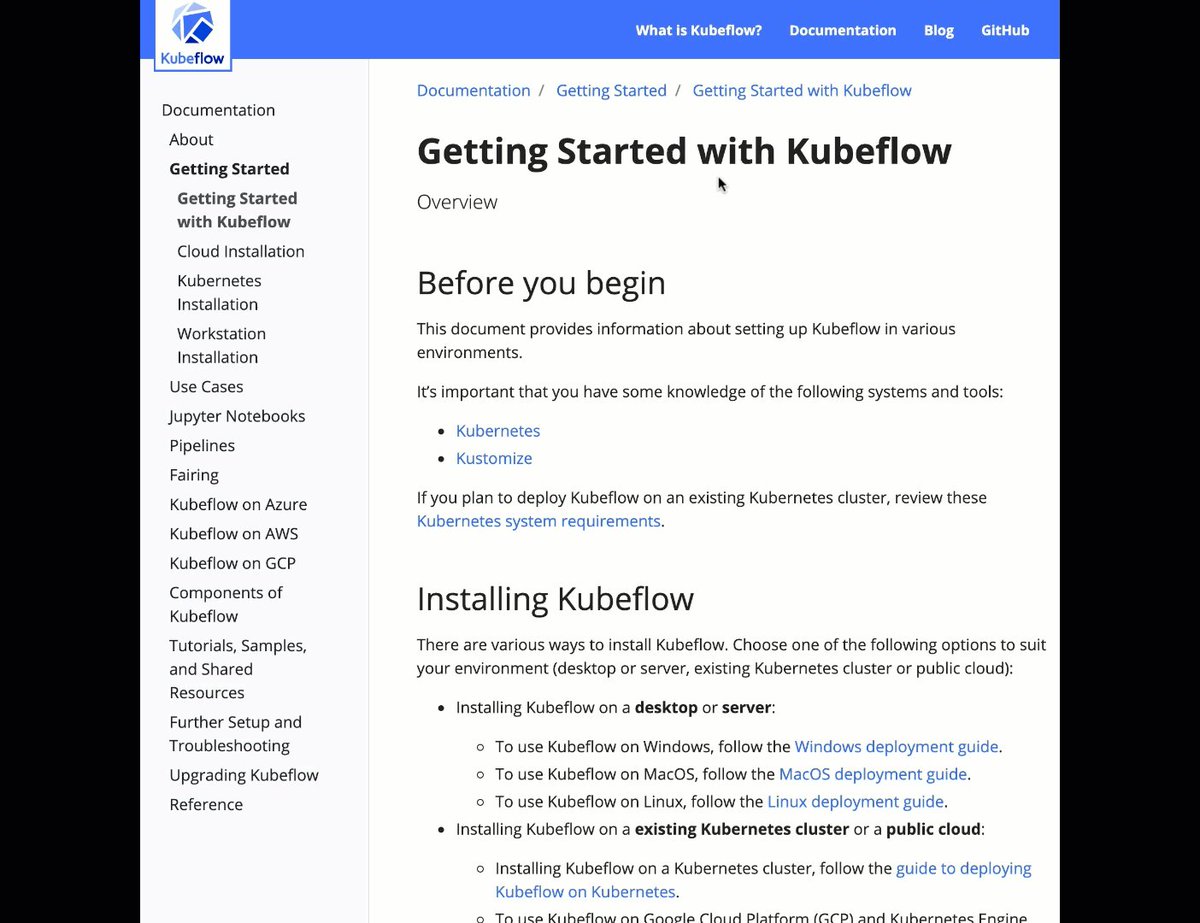